
November 3, 2024
AI in Architecture
Artificial Intelligence and computer vision are revolutionizing the architecture industry. From generative design to heritage restoration, let's explore how AI and CV are reshaping architecture
READ MORE

November 14, 2024
AI in driverless cars
It's likely that after a couple of decades, humans will be banned from driving cars altogether: because AI in smart cars will handle it better.
READ MORE
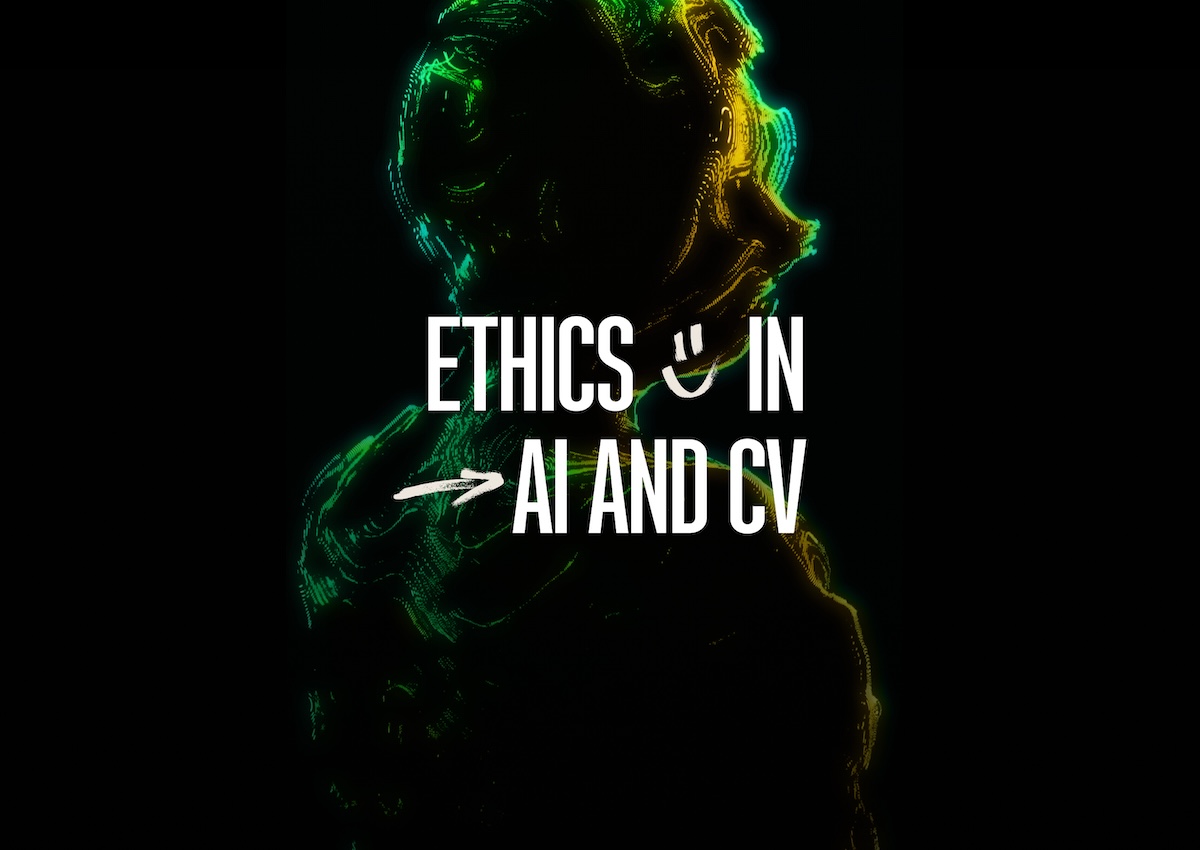
October 17, 2024
Ethics in artificial intelligence and computer vision
Smart machines are making decisions in people's life death and taxes, — and let's be honest, they don't always do it well or transparently
READ MORE
.png)
October 4, 2024
Artificial intelligence and computer vision in education
How smart machines make learning easier and cheating harder
READ MORE
.jpg)
September 27, 2024
Robotics and agriculture
Let's explore how AI for agriculture improving the lives of animals, plants, and humans alike
READ MORE
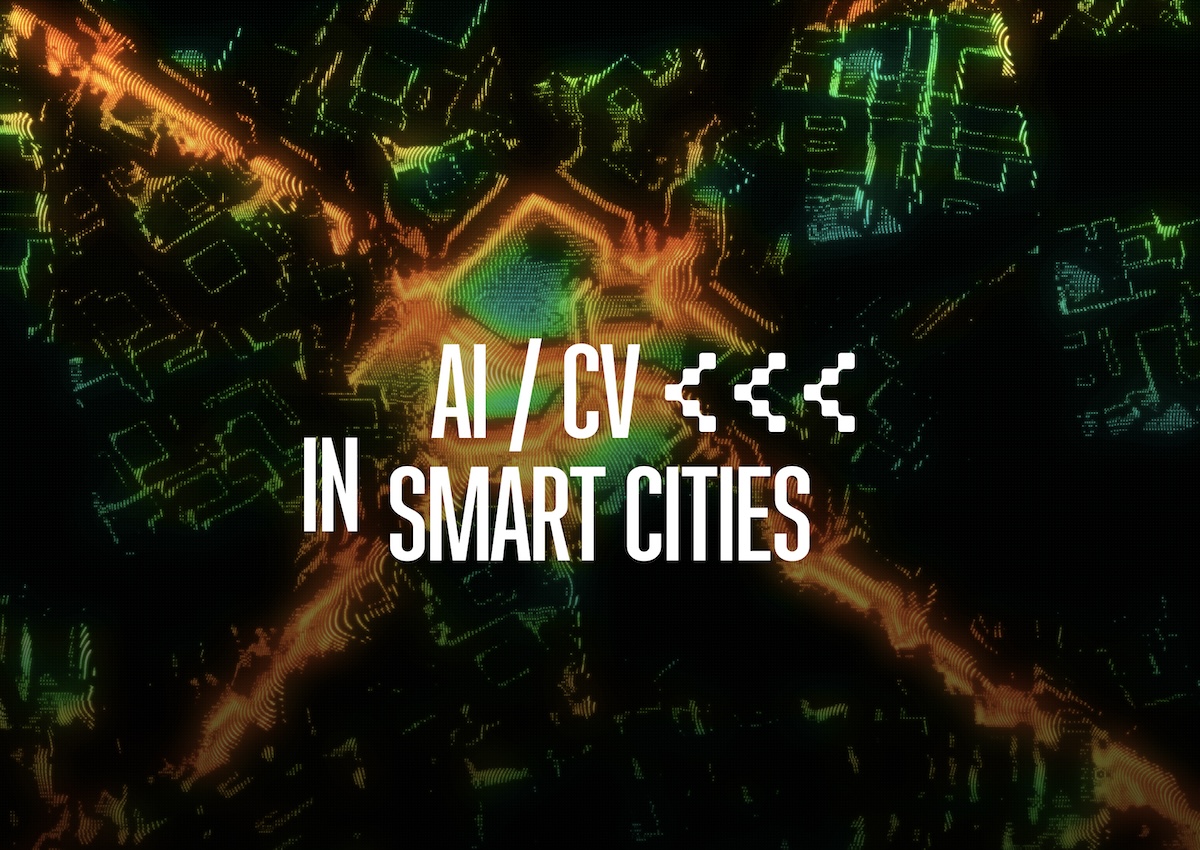
September 11, 2024
Computer vision and artificial intelligence in smart cities
Let's talk about how cities are getting smarter, greener, safer, more dynamic — right now
READ MORE
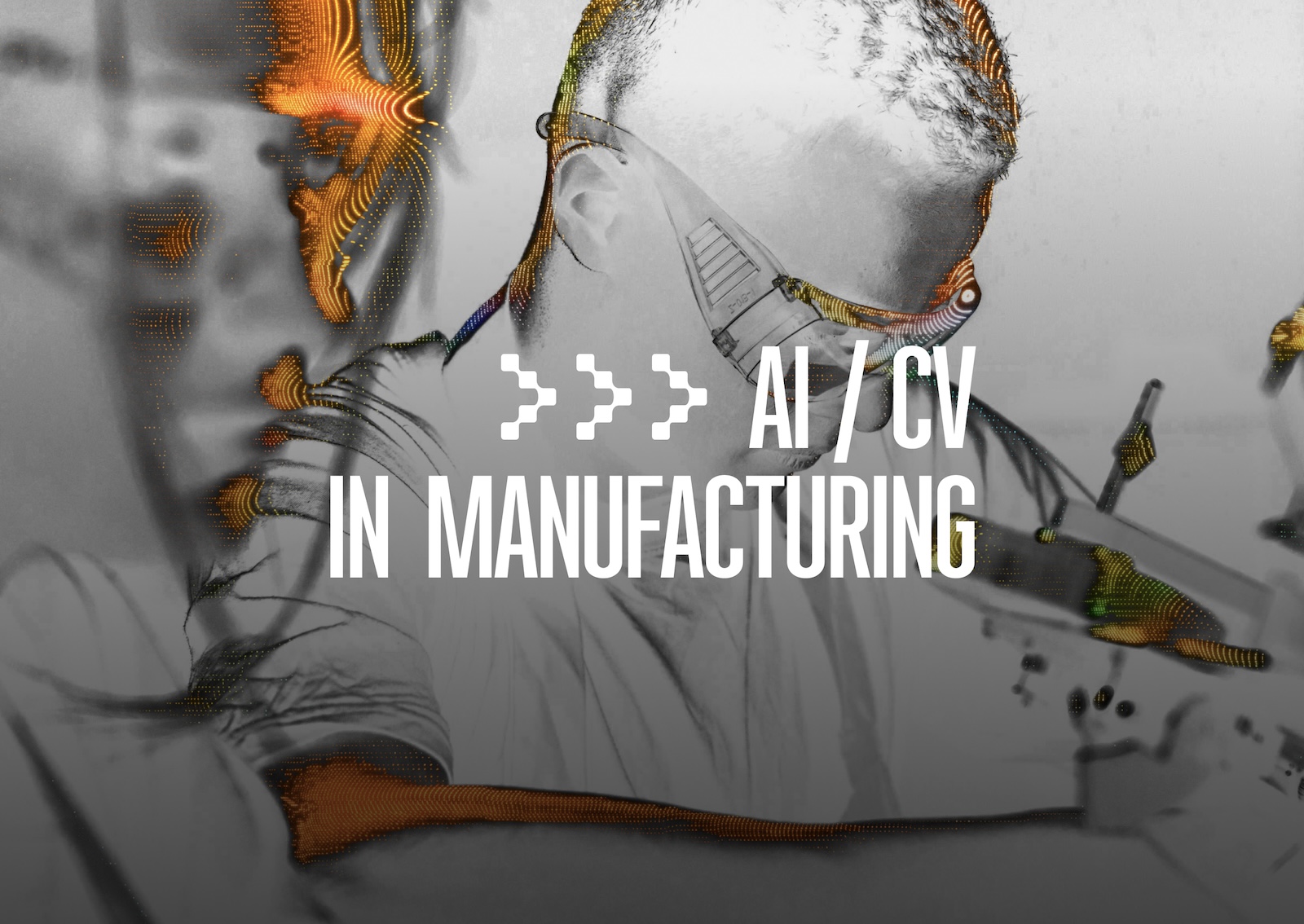
August 26, 2024
Computer vision and artificial intelligence in manufacturing
A few examples of how modern technology makes it possible to produce things faster, cheaper — and more environmentally friendly
READ MORE
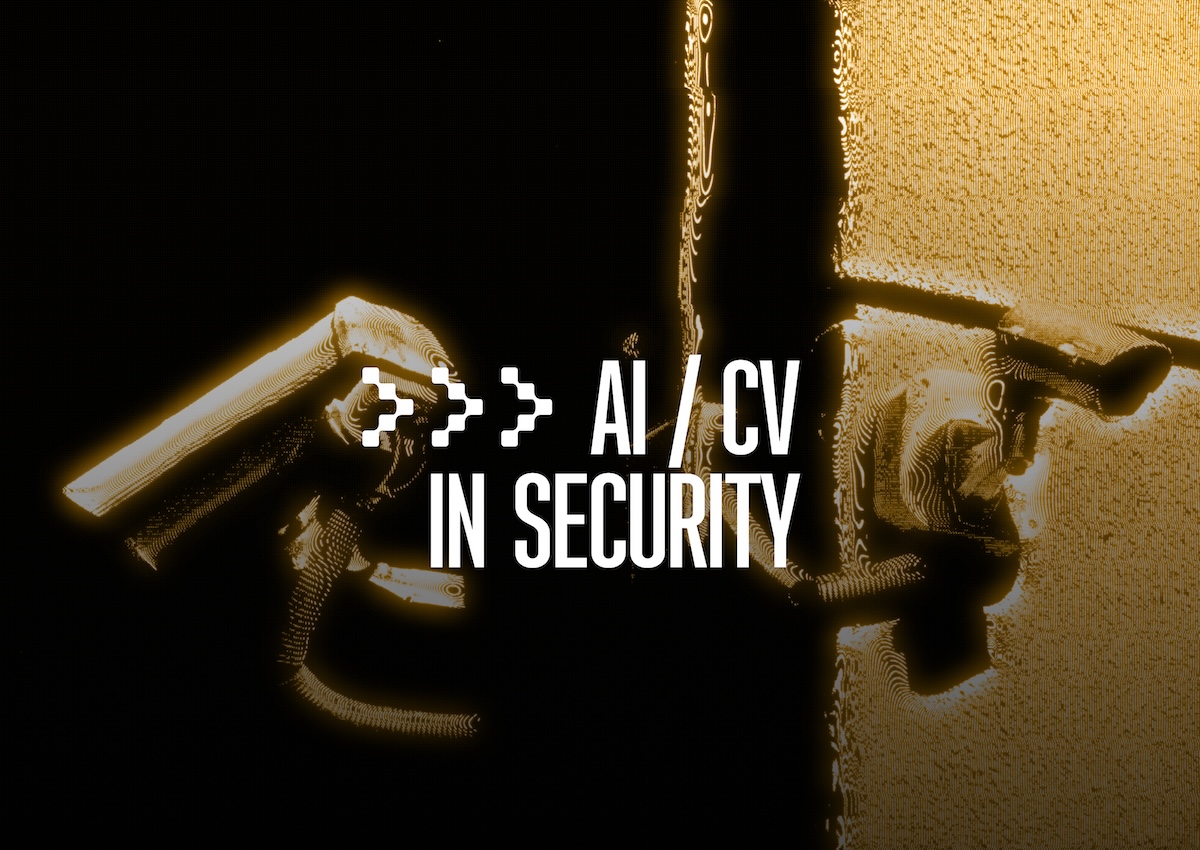
August 1, 2024
Computer vision and AI for security
Smart machines don't just heal, work in warehouses and stores — they also help make our world a safer place. Let us tell you how!
READ MORE
.jpg)
July 24, 2024
AI, AR, and VR — in Retail
AR (Augmented Reality) and VR (Virtual Reality) are often associated with gaming. However, these technologies hold significant potential in warehouse management.
READ MORE

July 18, 2024
AR and VR in Healthcare
AI is already better at diagnosing diseases, can perform minimal intervention surgeries — and make sure patients get better faster. Let's take a look at how VR and AR in medicine is actually done.
READ MORE
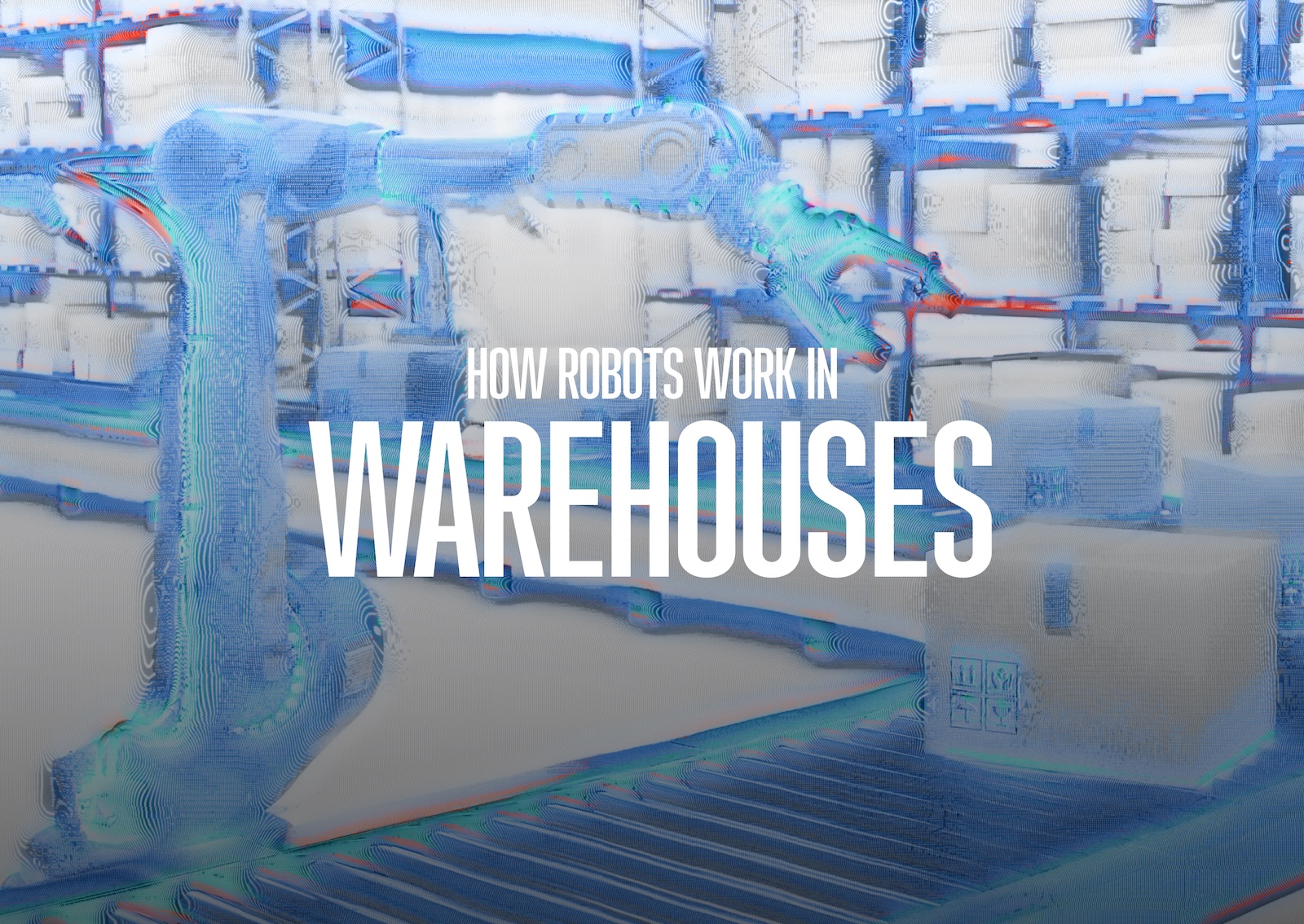
July 10, 2024
Artificial intelligence in warehouse
Amazon has about 1 million robots working in its warehouses alone — far more than human employees.
READ MORE
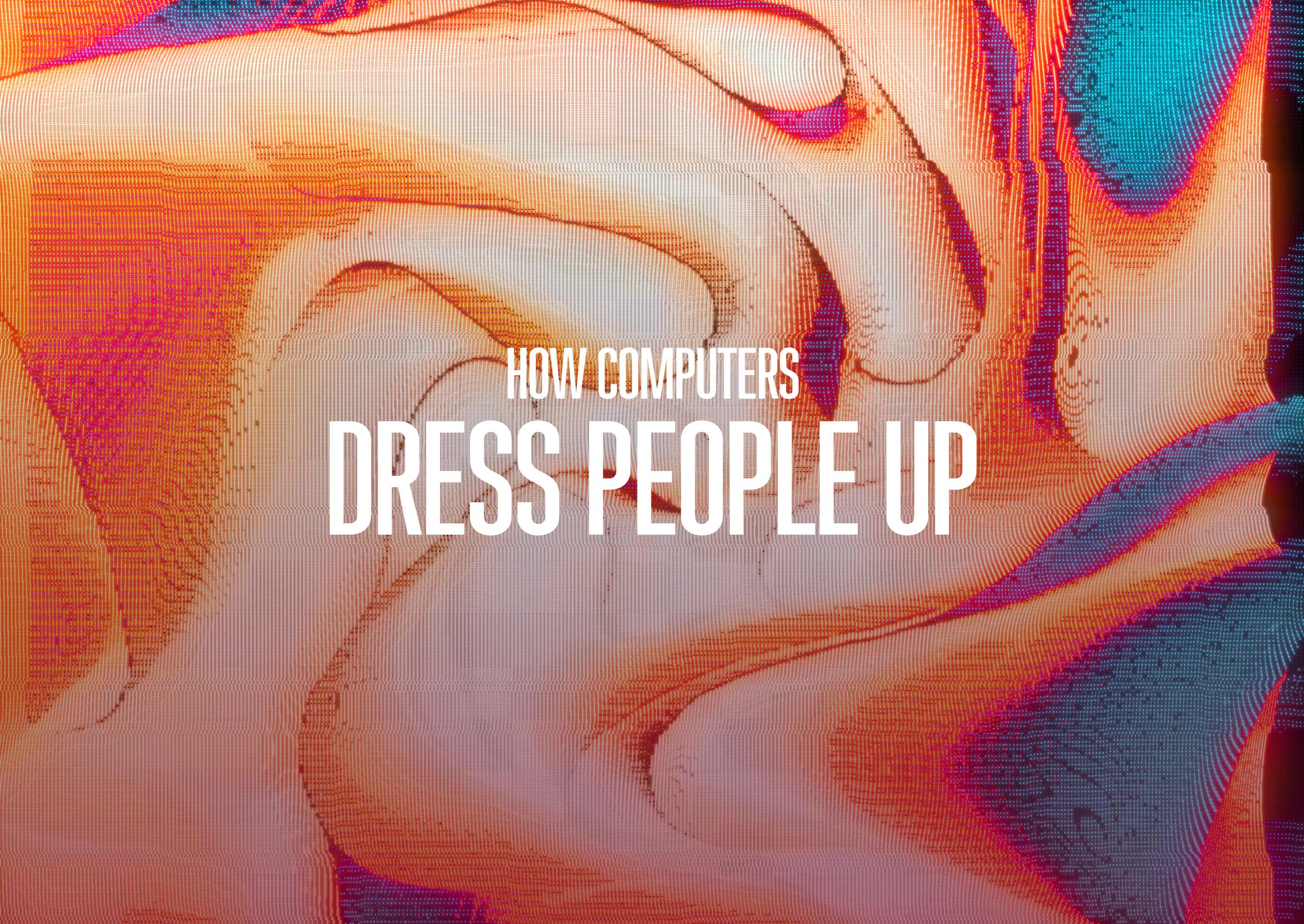
June 27, 2024
AI in Fashion
Everyone wants clothes that fit better and cost less.
READ MORE
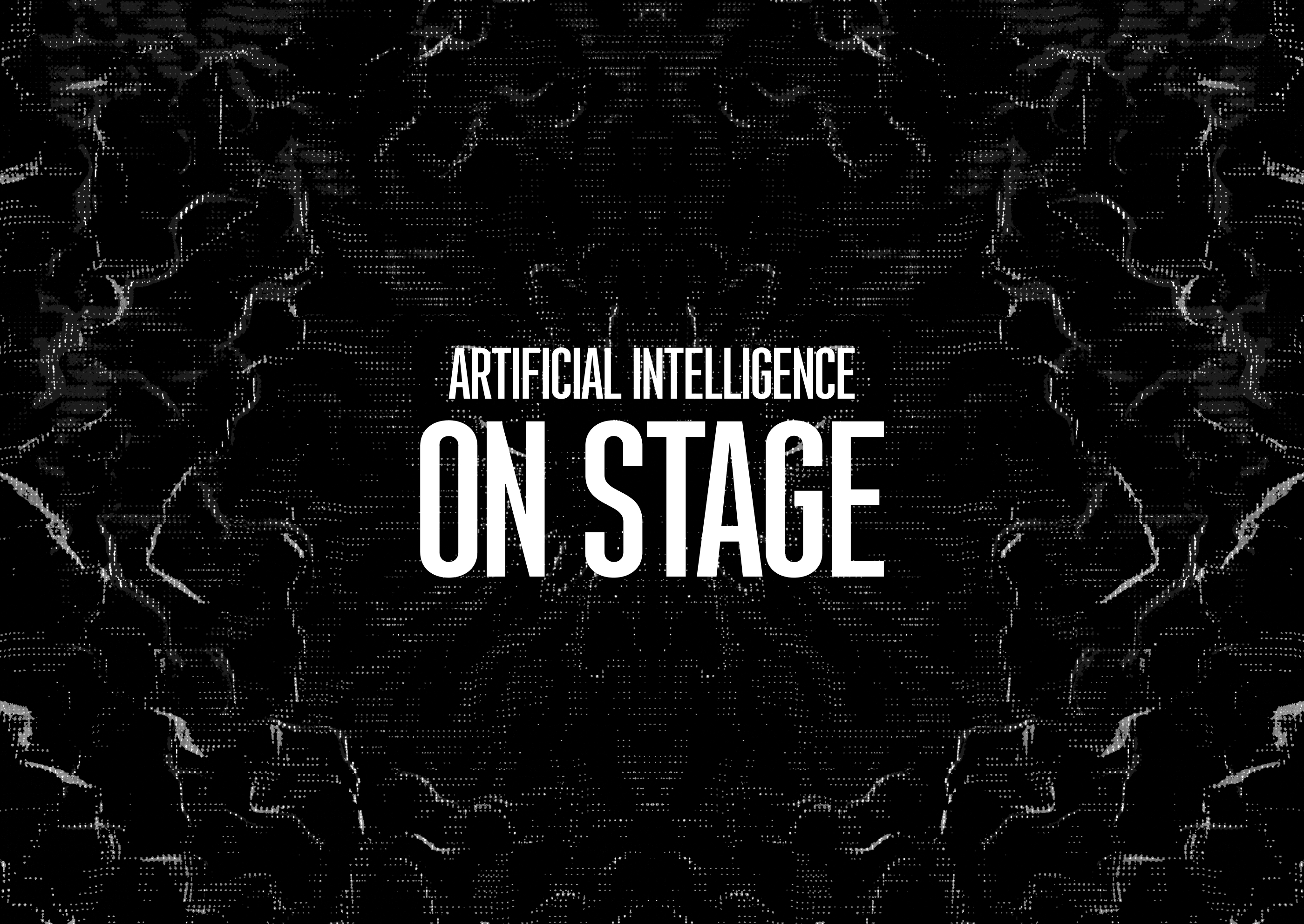
June 23, 2024
Artificial intelligence and computer vision — behind the microphone and on the stage
Robots can't do the dishes or clean our house yet, but they can already create a symphony.
READ MORE
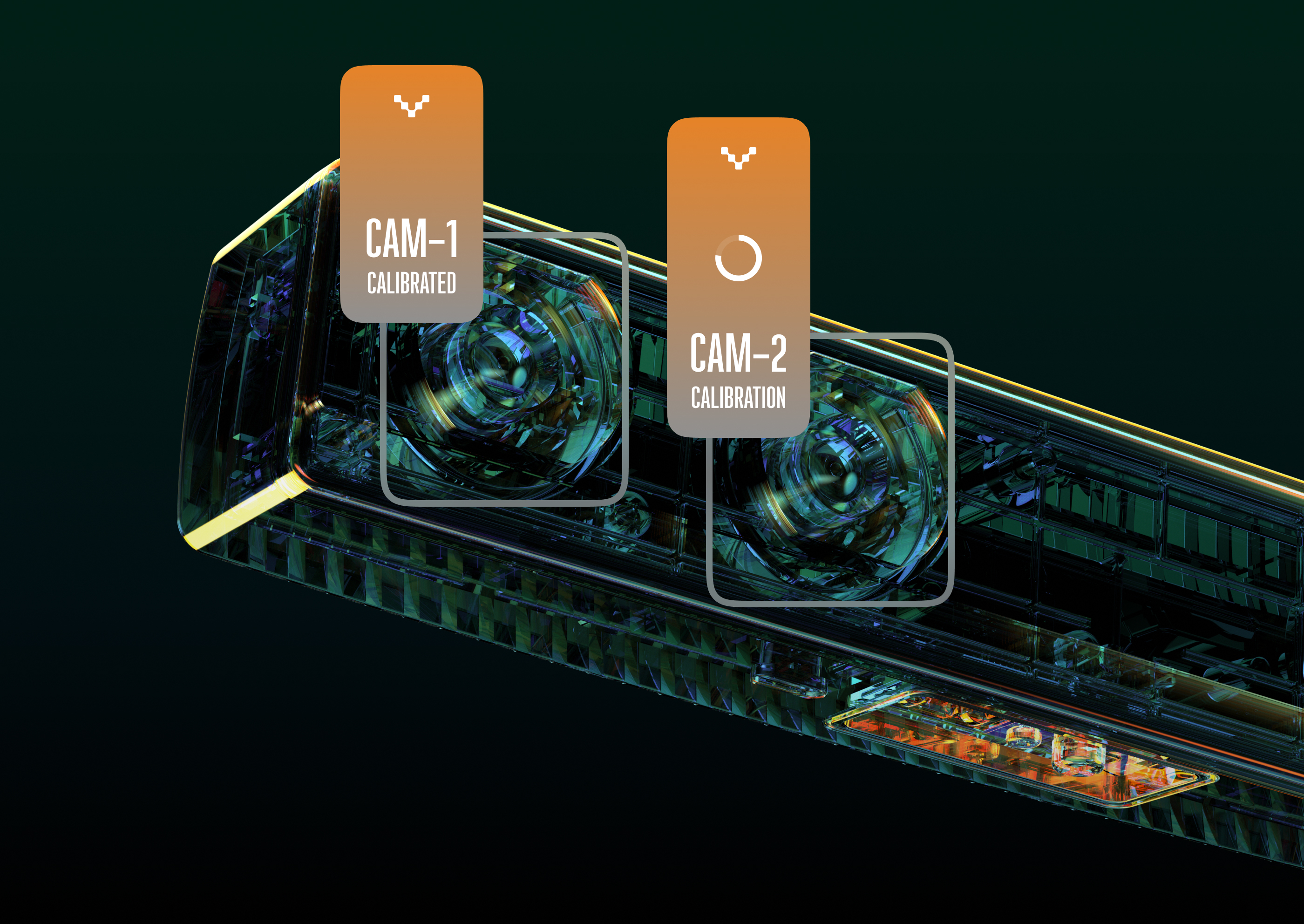
June 12, 2024
Why it's important to calibrate multiple cameras — and how to do it right
In the previous article, we talked about the importance of the camera calibration process, which is employed by computer vision and machine learning algorithms. We discussed how to do this by placing a pattern or, in some cases, using surrounding objects as a pattern in the camera's field of view. But if there is more than one camera, things get complicated!
READ MORE
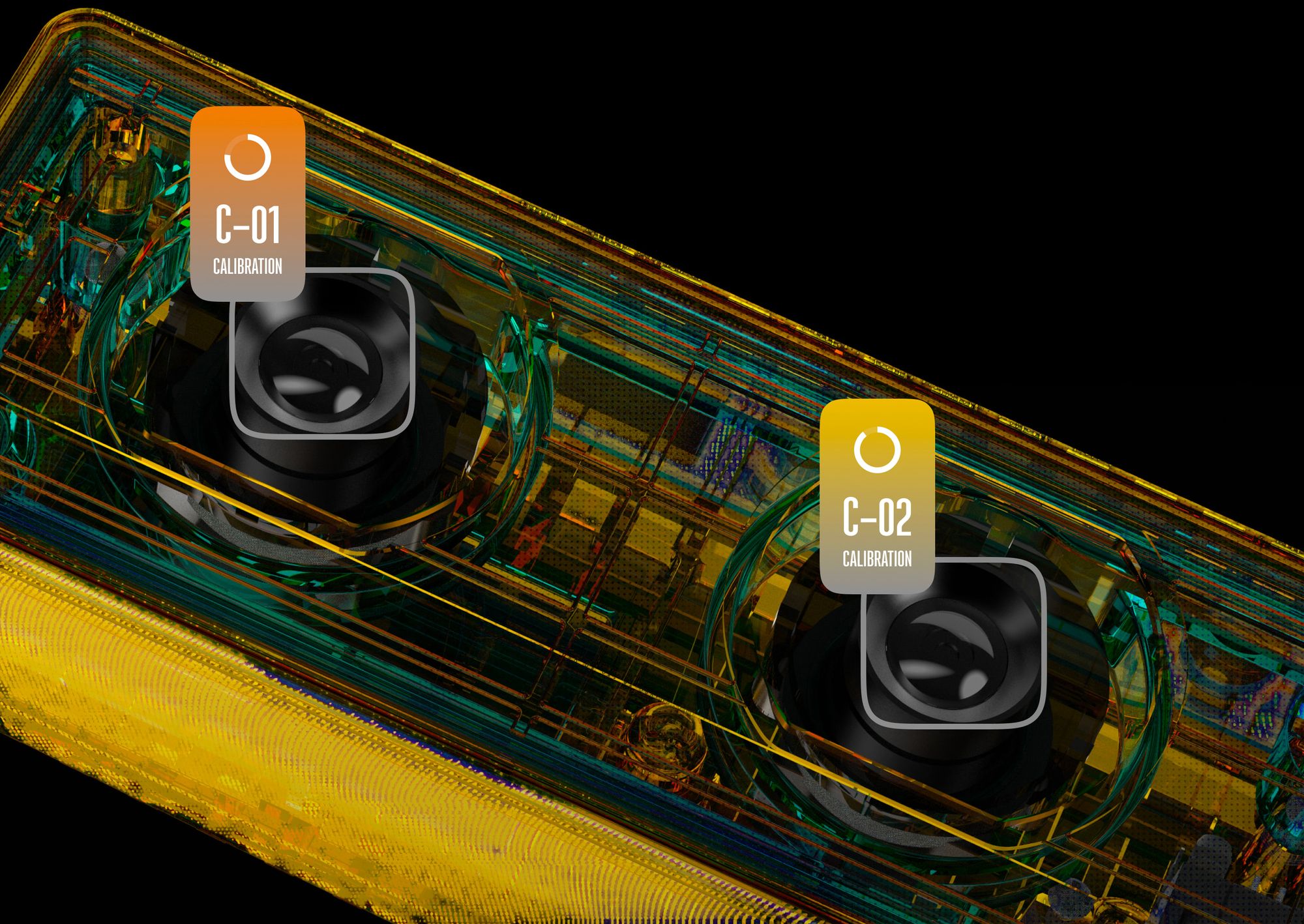
May 31, 2024
Why camera calibration is so important in computer vision
The cameras are used to capture the world around us. But frames are just representations of the world, and the actual relationship between the flat image and the real thing is not always obvious. In order to extract meaningful insights about the real objects from the images, developers and users of computer vision systems need to understand camera characteristics — with the help of the calibration solutions
READ MORE
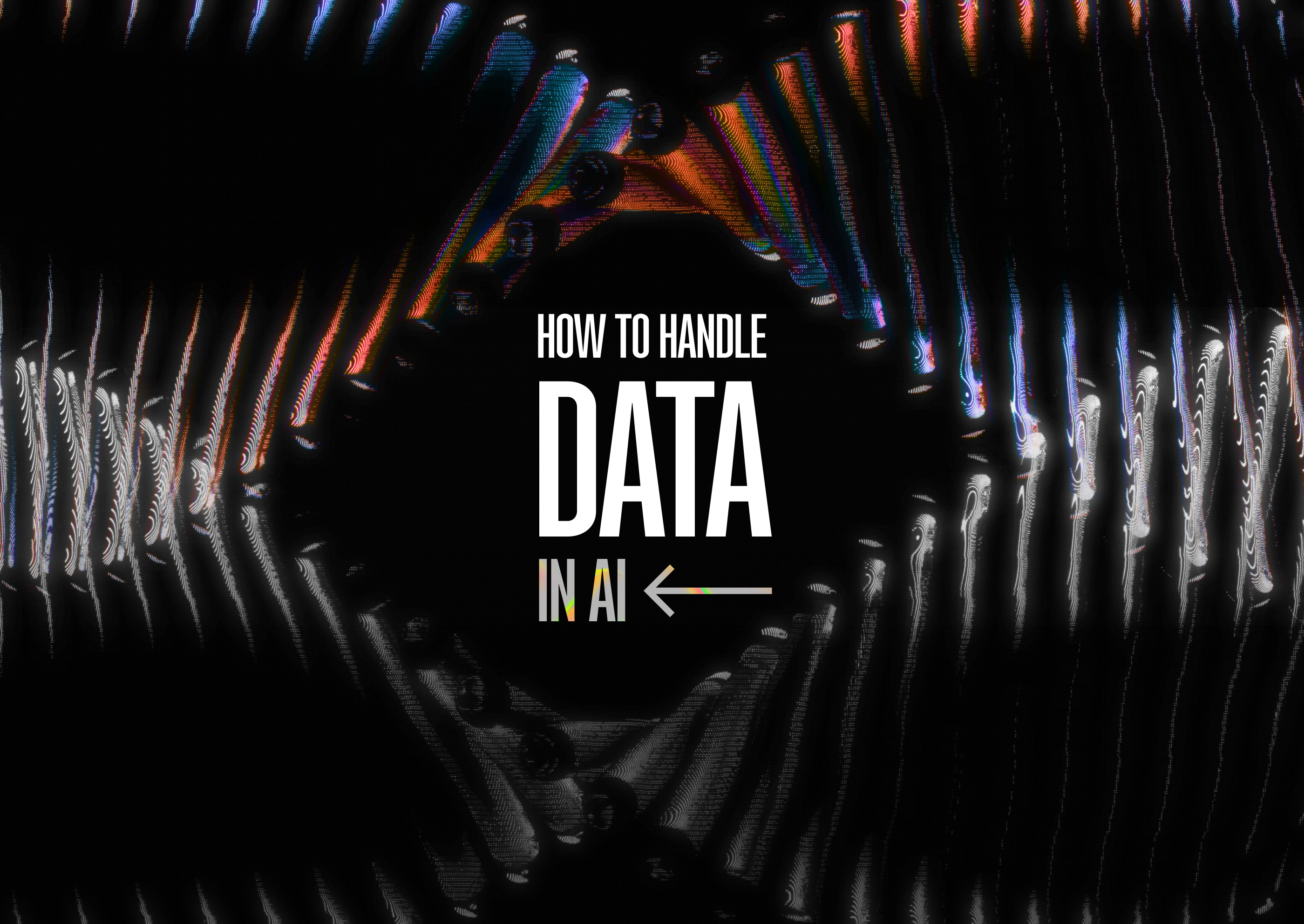
May 24, 2024
How to handle data in AI — Q&A
Without the suitable and well-organized data, one is bound to face the dreaded "Garbage in, Garbage out" problem — even the most perfect AI model will perform poorly if the input data is poor. Here's how to refine the "fuel" that powers your advanced AI engine!
READ MORE
.jpg)
May 15, 2024
AI in football
Football is not only the most popular sport, watched by more than 4 billion people around the world — it is also a huge market. Some of the strongest clubs in Europe are businesses with annual revenues of $8 billion and more than 100,000 employees in different countries. Football is also a competition field for the latest technologies in computer vision and artificial intelligence. Let's take a look at what AI and CV are doing in football!
READ MORE
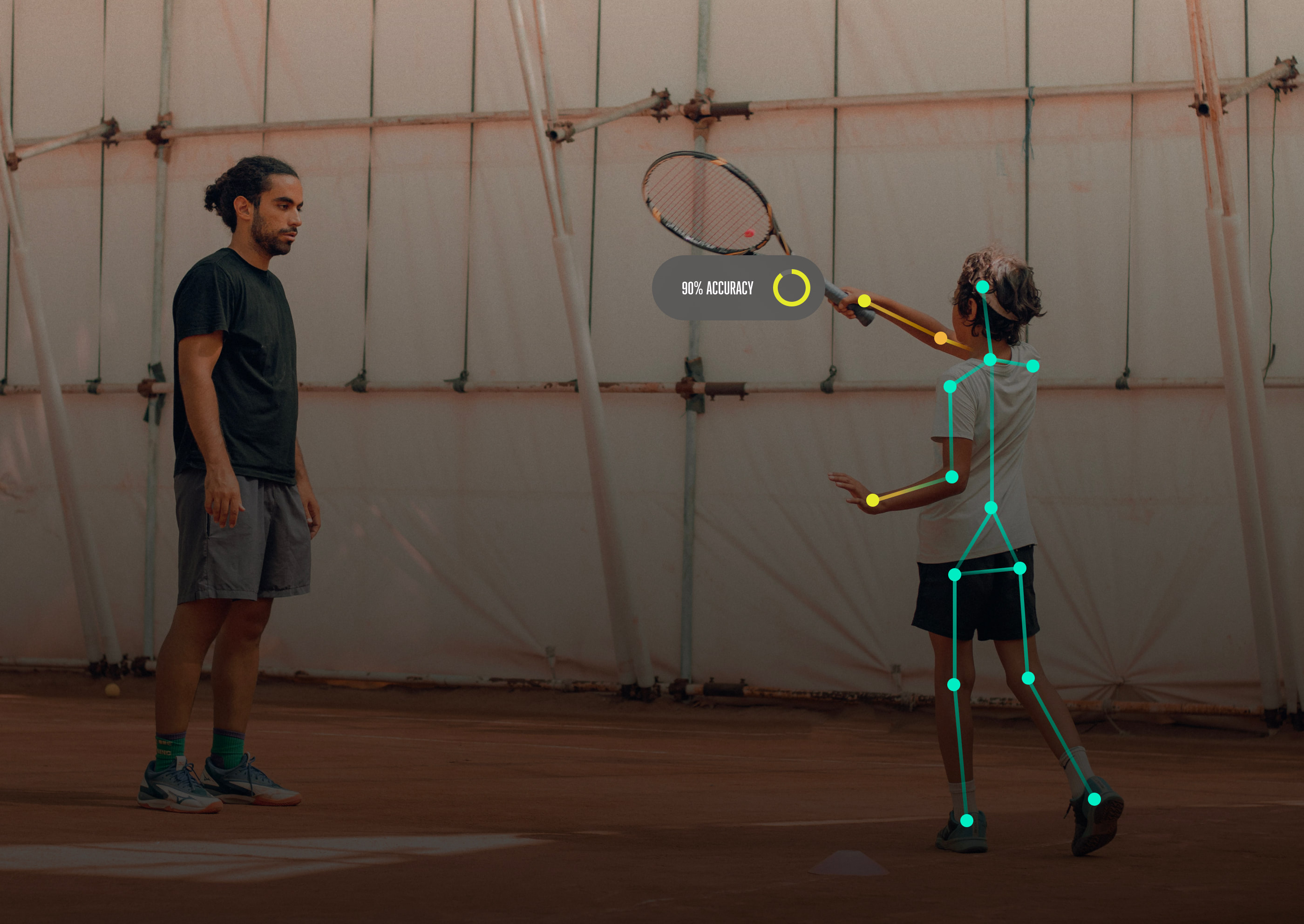
May 7, 2024
Computer Vision in Sports: People Train and Compete — Machines Watch and Help
At the upcoming 2024 Olympic Games in Paris, the world will see the most advanced AI and computer vision systems for sports developed by Intel. These systems will not only help capture athletic performance with millimeter and millisecond accuracy but also create 3D models of athletes for replays and analyzing complex situations. The data and models will be available to both referees and spectators.
Artificial intelligence and computer vision systems in sports are no longer a high-tech novelty but an everyday reality. People train, challenge, and watch others compete — and hundreds of tech companies are helping to make it safer and more efficient. And more fun, too!
READ MORE

April 16, 2024
Which GPUs are the most relevant for Computer Vision
In the field of CV, selecting the appropriate hardware can be tricky due to the variety of usable machine-learning models and their significantly different architectures. Today’s article explores the criteria for selecting the best GPU for computer vision, outlines the GPUs suited for different model types, and provides a performance comparison to guide engineers in making informed decisions.
READ MORE
.jpg)
April 12, 2024
Digest 19 | OpenCV AI Weekly Insights
Explore OpenCV's distribution for Android, iPhone LiDAR depth estimation, simplified GPT-2 model training by Andrej Karpathy, and Apple's ReALM system, promising enhanced AI interactions.
READ MORE
.jpg)
June 7, 2024
How To Budget For a Computer Vision AI Solution? Part 2 | Software
This article offers a comprehensive guide to budgeting for computer vision AI solutions, focusing on critical software factors. It covers key aspects such as task analysis, data collection, annotation, model development, and pipeline implementation, providing insights for making informed financial decisions.
READ MORE
.jpg)
April 11, 2024
OpenCV For Android Distribution
The OpenCV.ai team, creators of the essential OpenCV library for computer vision, has launched version 4.9.0 in partnership with ARM Holdings. This update is a big step for Android developers, simplifying how OpenCV is used in Android apps and boosting performance on ARM devices.
READ MORE
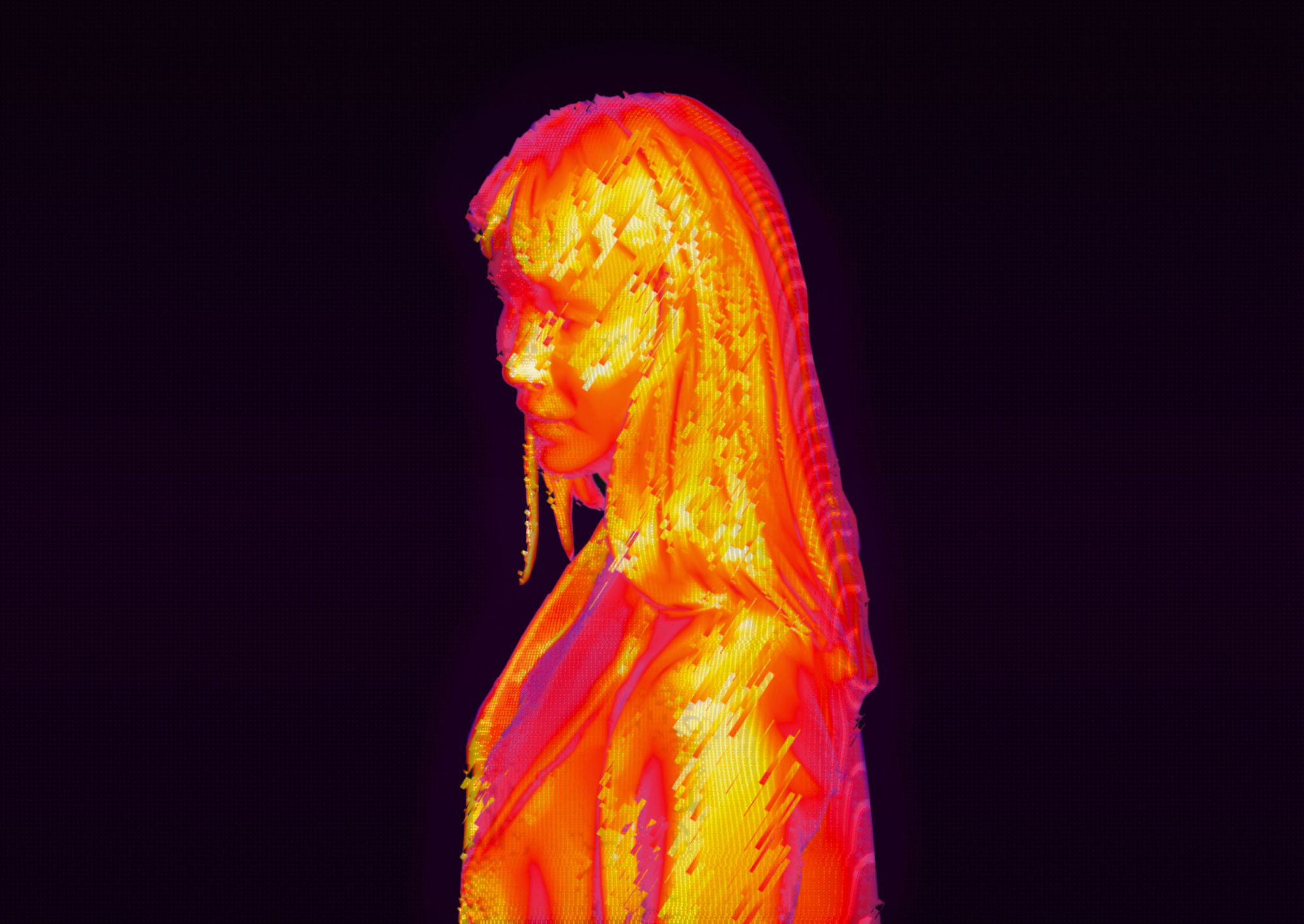
April 4, 2024
Depth estimation technology in iPhones
The article examines the iPhone's LiDAR technology: its depth measurement for improved photography, augmented reality, and navigation. LiDAR contributes to digital experiences by mapping environments.
READ MORE
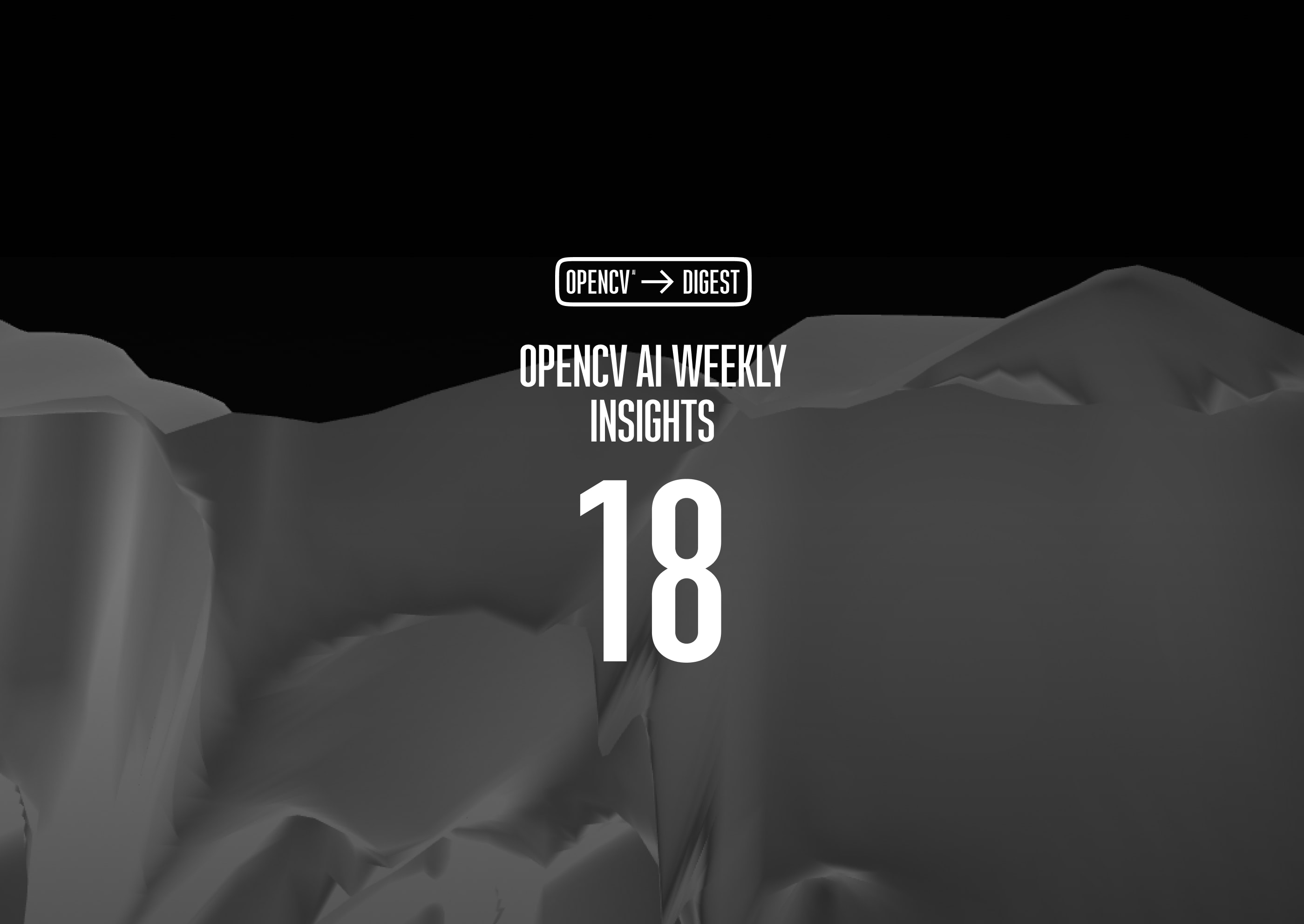
April 2, 2024
Digest 18 | OpenCV AI Weekly Insights
Discover the latest in AI: Hume AI's EVI introduces emotional intelligence to technology, OpenAI's Voice Engine offers realistic voice cloning with ethical safeguards, RadSplat pushes VR rendering speeds, and xAI's Grok-1.5 advances in understanding complex contexts.
READ MORE
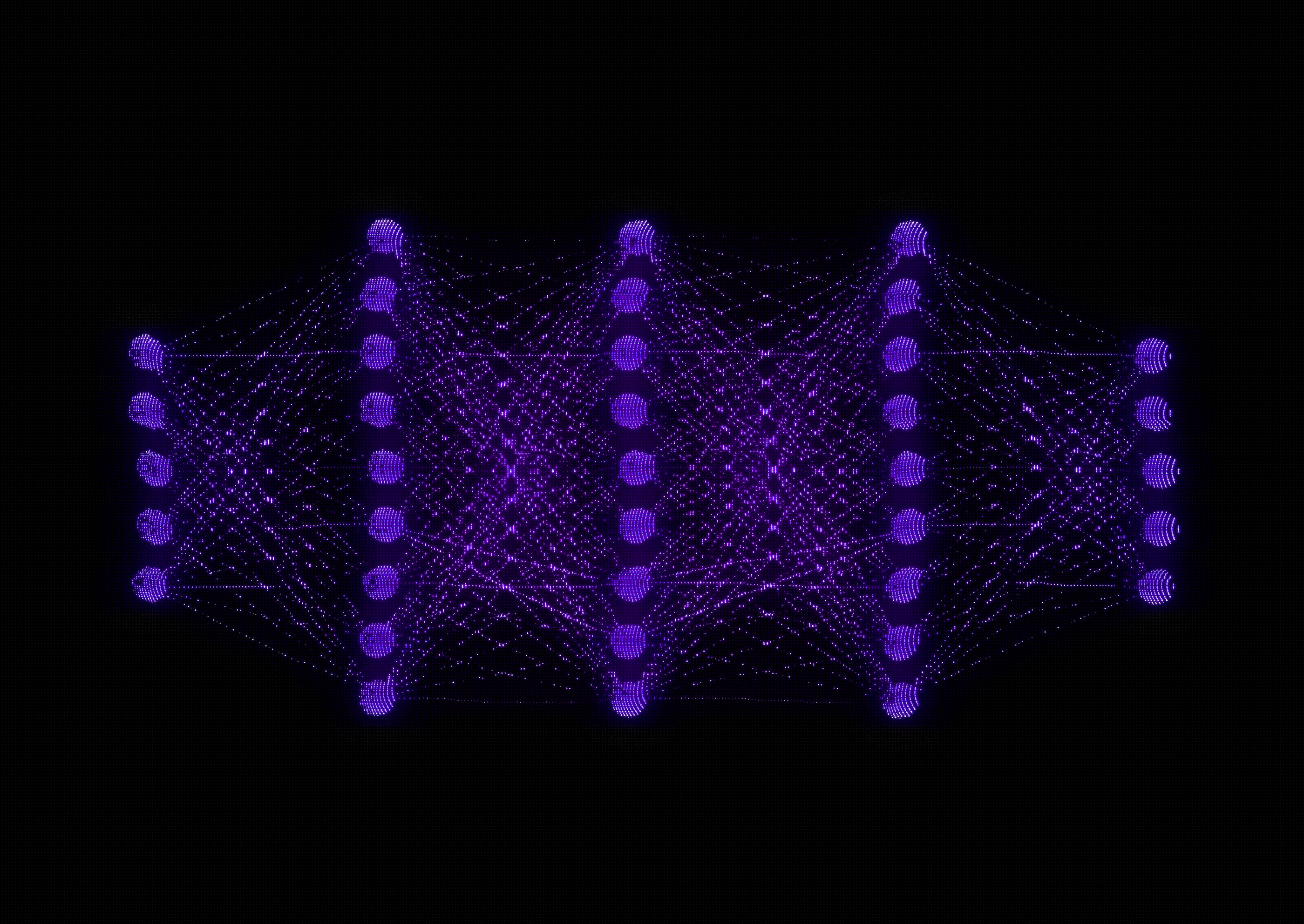
March 21, 2024
How To Train a Neural Network with less GPU Memory: Reversible Residual Networks Review
Explore how reversible residual networks save GPU memory during neural network training.
READ MORE
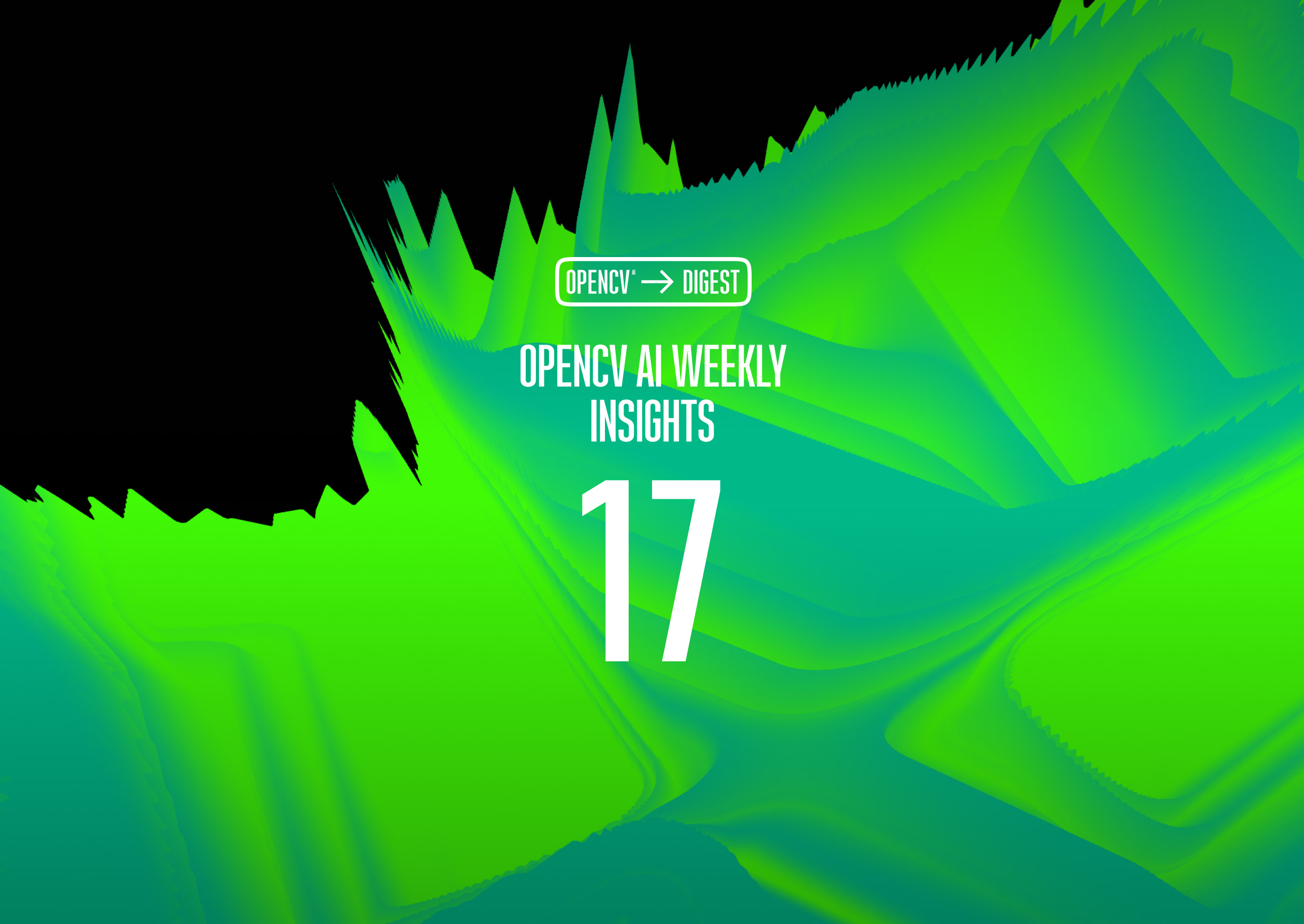
March 20, 2024
Digest 17 | OpenCV AI Weekly Insights
Discover the latest in AI: Nvidia's groundbreaking Blackwell B200 GPU and GB200 superchips, Google's innovative VLOGGER AI that animates avatars from photos, insights into GPT-5 from Sam Altman, and Elon Musk's commitment to open AI with the release of Grok-1. Stay informed with our summary of these advancements.
READ MORE
.jpg)
March 7, 2024
Examples Of AI In Healthcare
Explore the impact of AI on healthcare, showcasing advances in drug development, robotic surgery, personalized medicine, disease detection, and wearable devices.
READ MORE

February 29, 2024
Computer Vision in Warehousing and Logistics
Explore the impact of computer vision in logistics, driving advancements in warehousing efficiency, accuracy, and customer satisfaction.
READ MORE
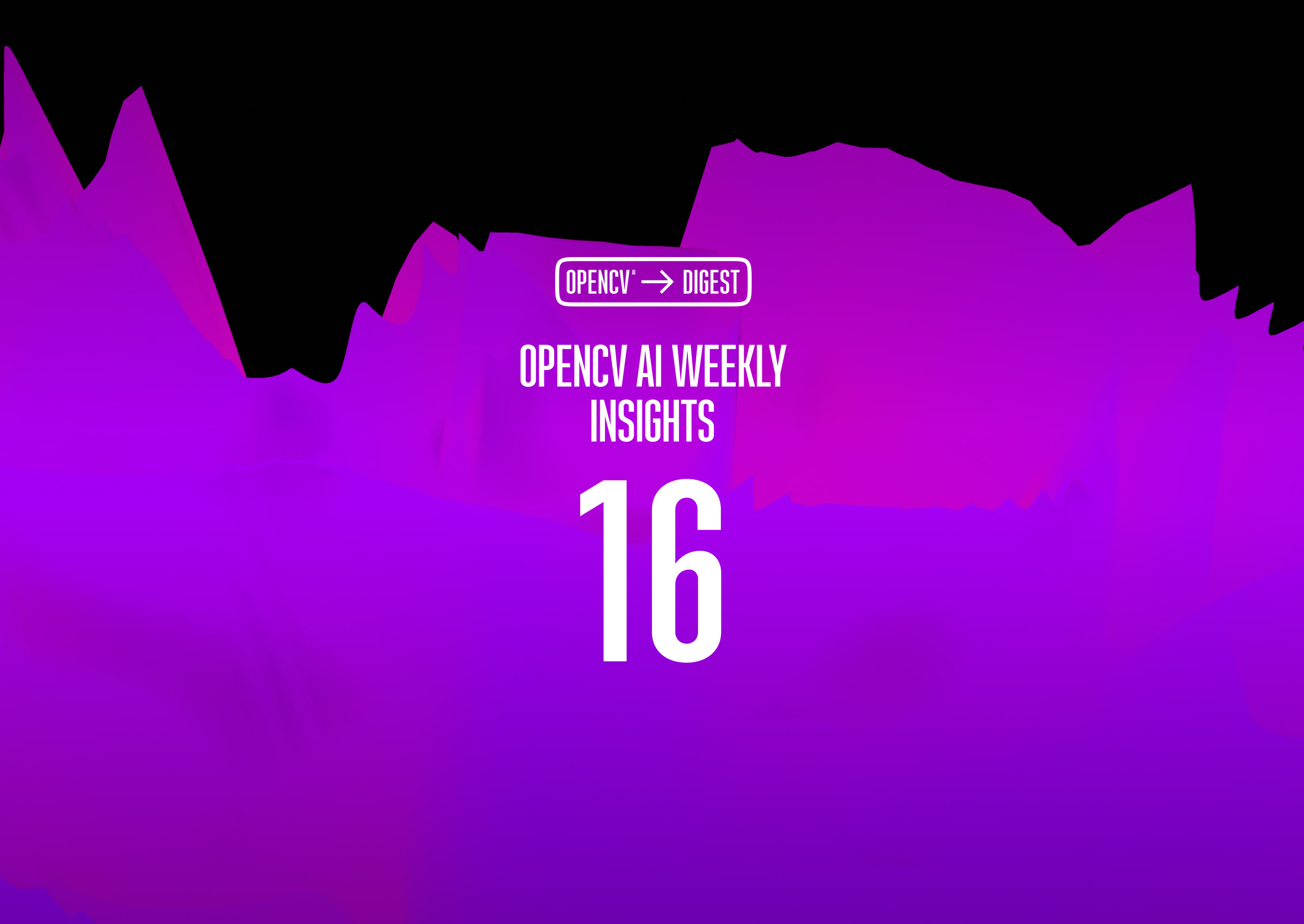
February 27, 2024
Digest 16 | OpenCV AI Weekly Insights
Check out this week's top AI news in our digest! Learn about OpenAI's new video-making AI, a brain chip that controls computers with thoughts, a better way to create digital art, and a big investment in robots that could take over tough jobs.
READ MORE

February 15, 2024
Understanding the AI Act Requirements for Product Companies
This article outlines the AI Act requirements and how they impact product companies.
READ MORE

February 22, 2024
Animal Behavior Recognition Using Machine Learning
Our article reviews key AI methods in animal behavior recognition and animal pose detection, showing their application in fields from neurobiology to veterinary medicine.
READ MORE
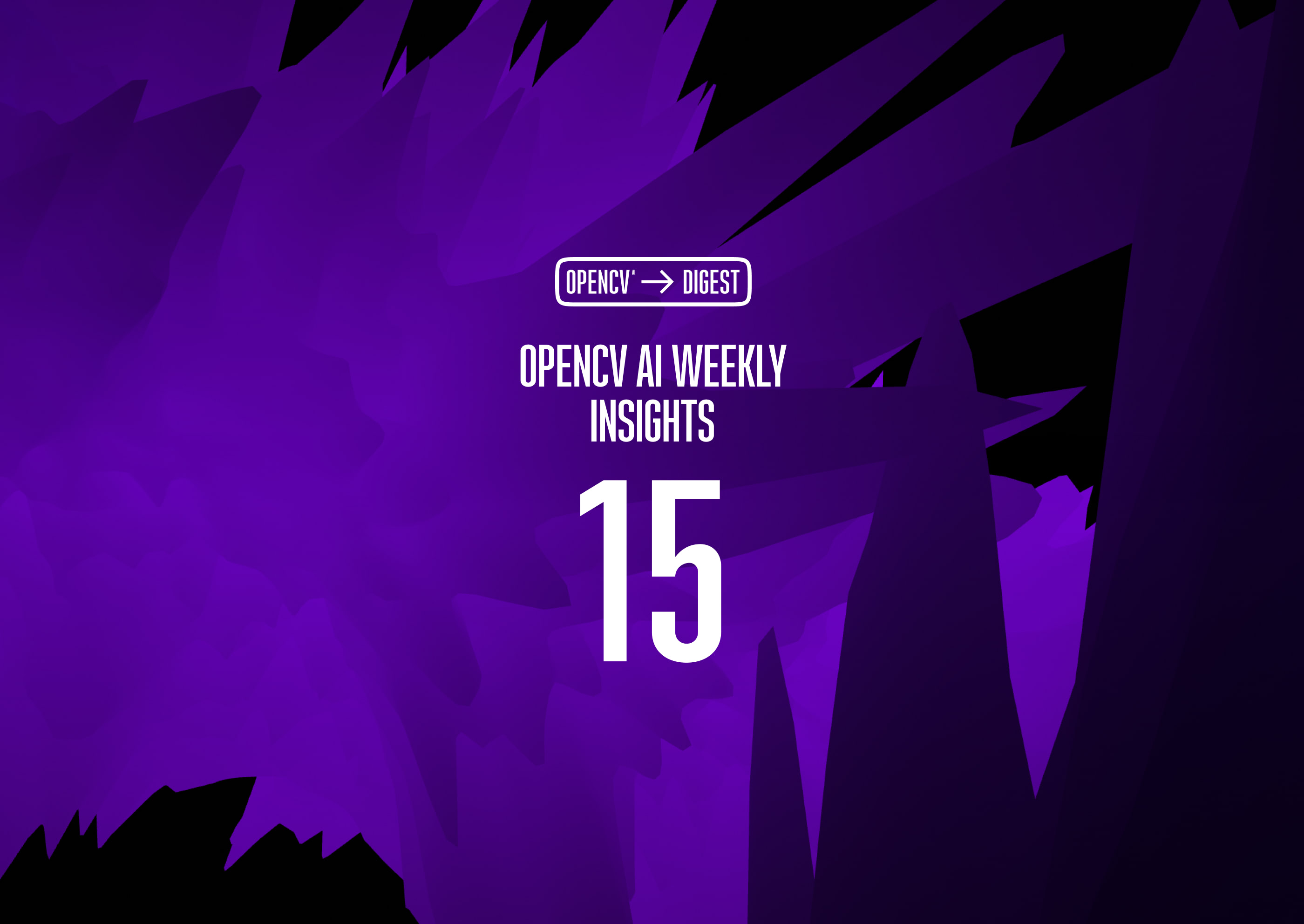
February 13, 2024
Digest 15 | OpenCV AI Weekly Insights
Keep at the forefront of technology and AI with our latest insights, featuring Adobe's pioneering fashion tech, Brilliant Labs' advanced AR glasses, and the groundbreaking Gemini Ultra by Google.
READ MORE
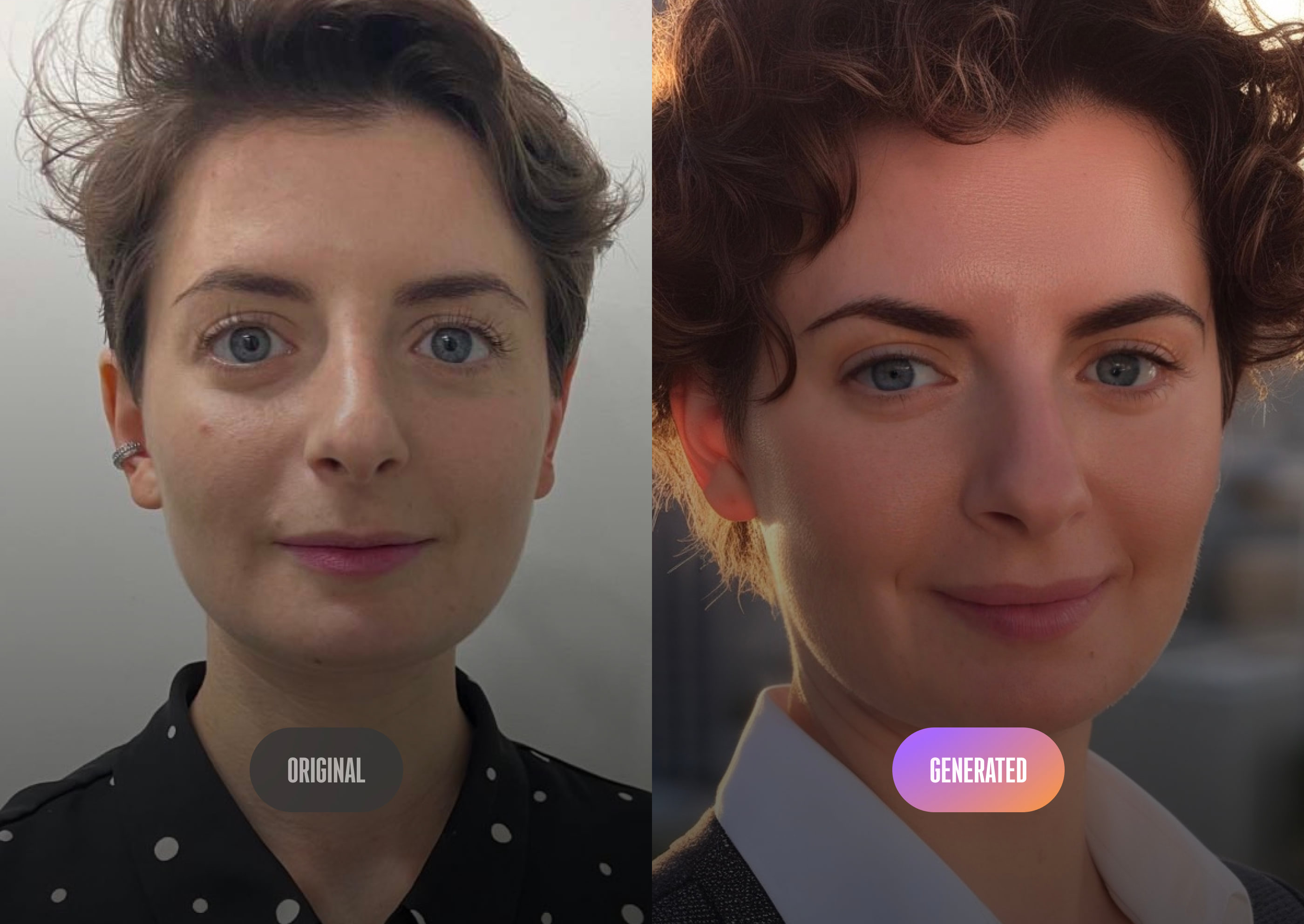
February 9, 2024
How Computer Vision Techniques Make People Look More Attractive
Explore the capabilities of computer vision techniques for facial enhancement in our thorough review.
READ MORE
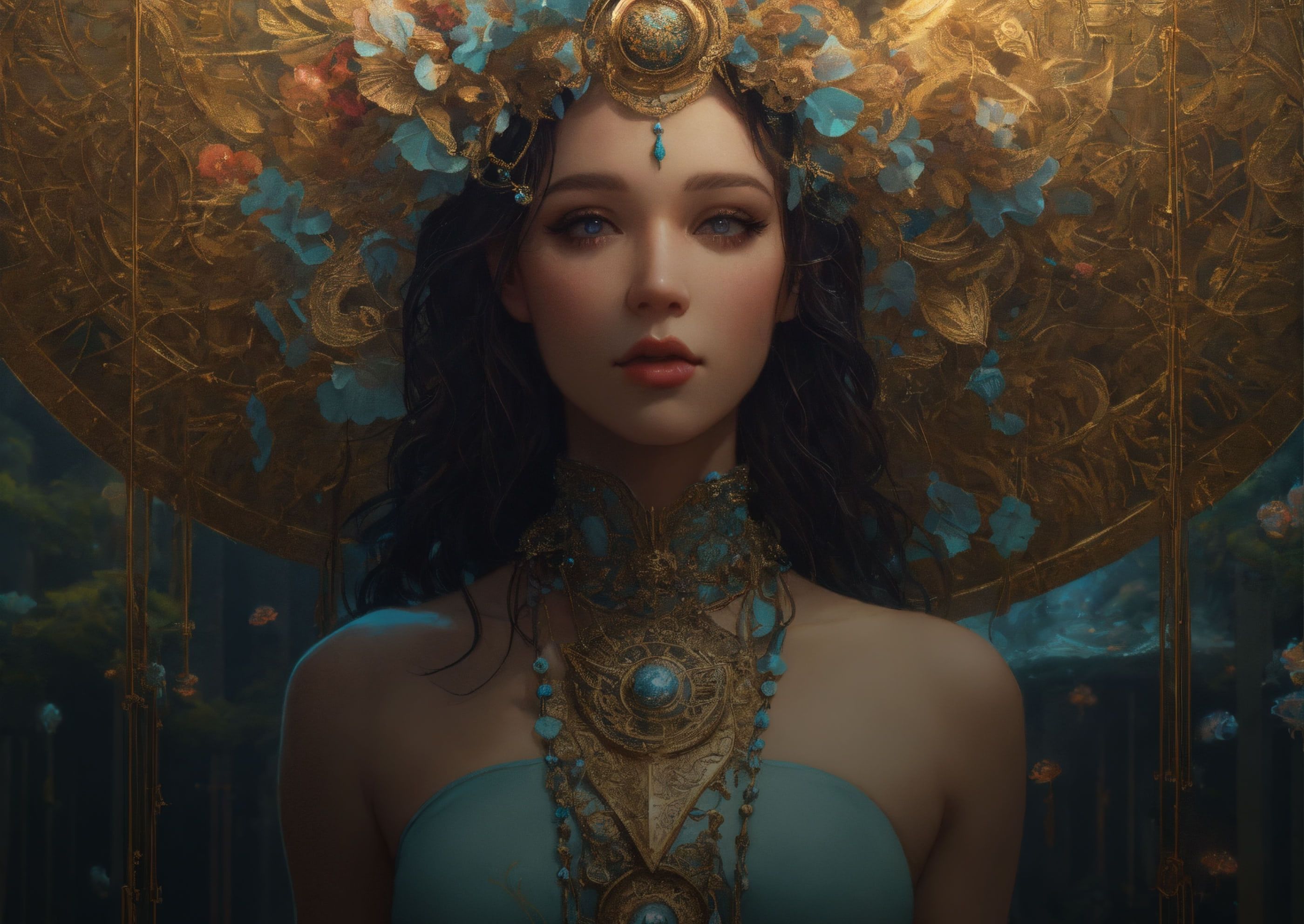
February 2, 2024
AI and Art: The Brush of the Future
Dive into the intersection of art, technology, and AI with our latest exploration, where we review how generative AI tools like Stable Diffusion create art.
READ MORE
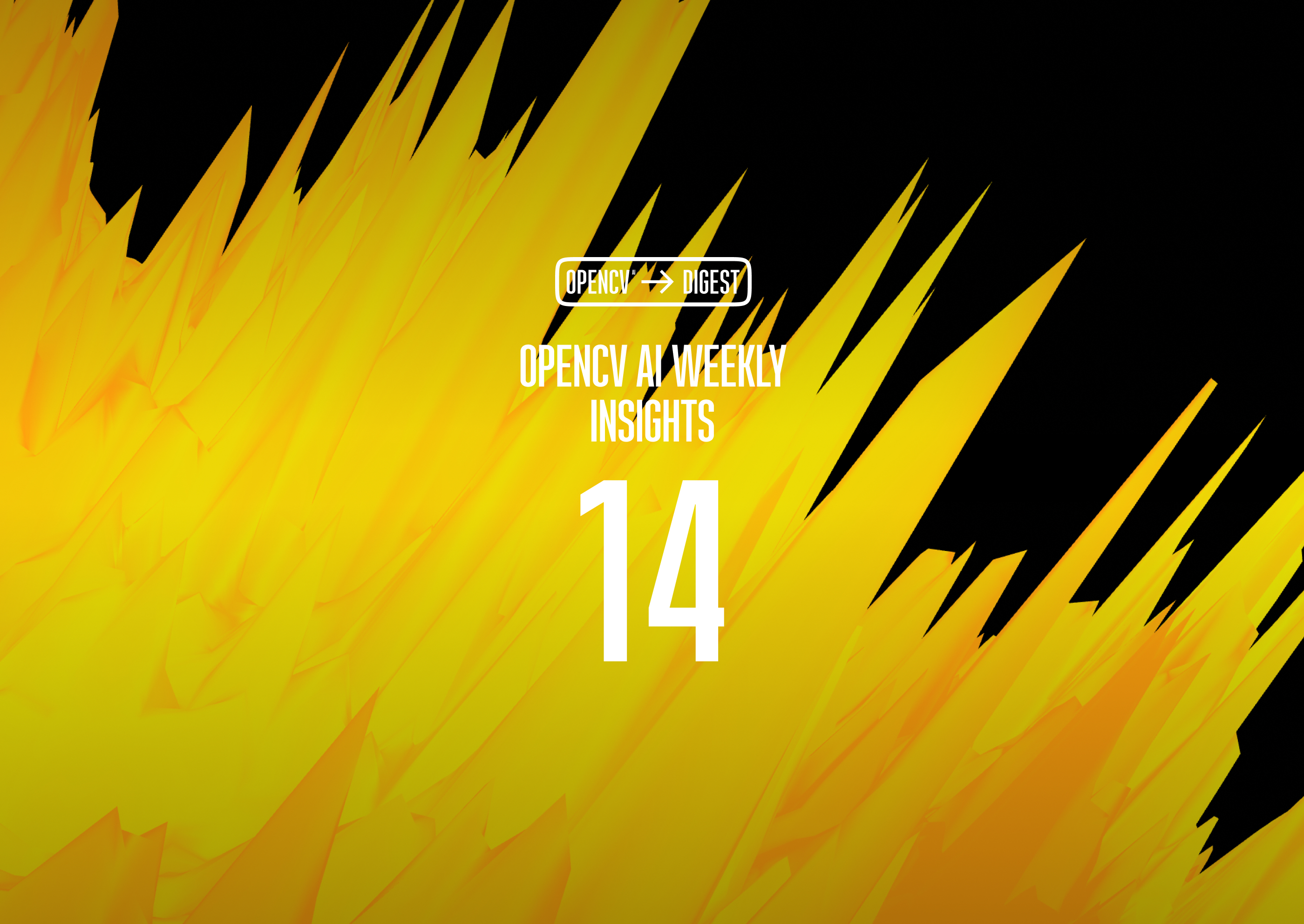
January 30, 2024
Digest 14 | OpenCV AI Weekly Insights
Read about the latest on the multi-GPT ChatGPT update, groundbreaking developments in humanoid robotics, and existing dream technology – delve into the full digest now.
READ MORE
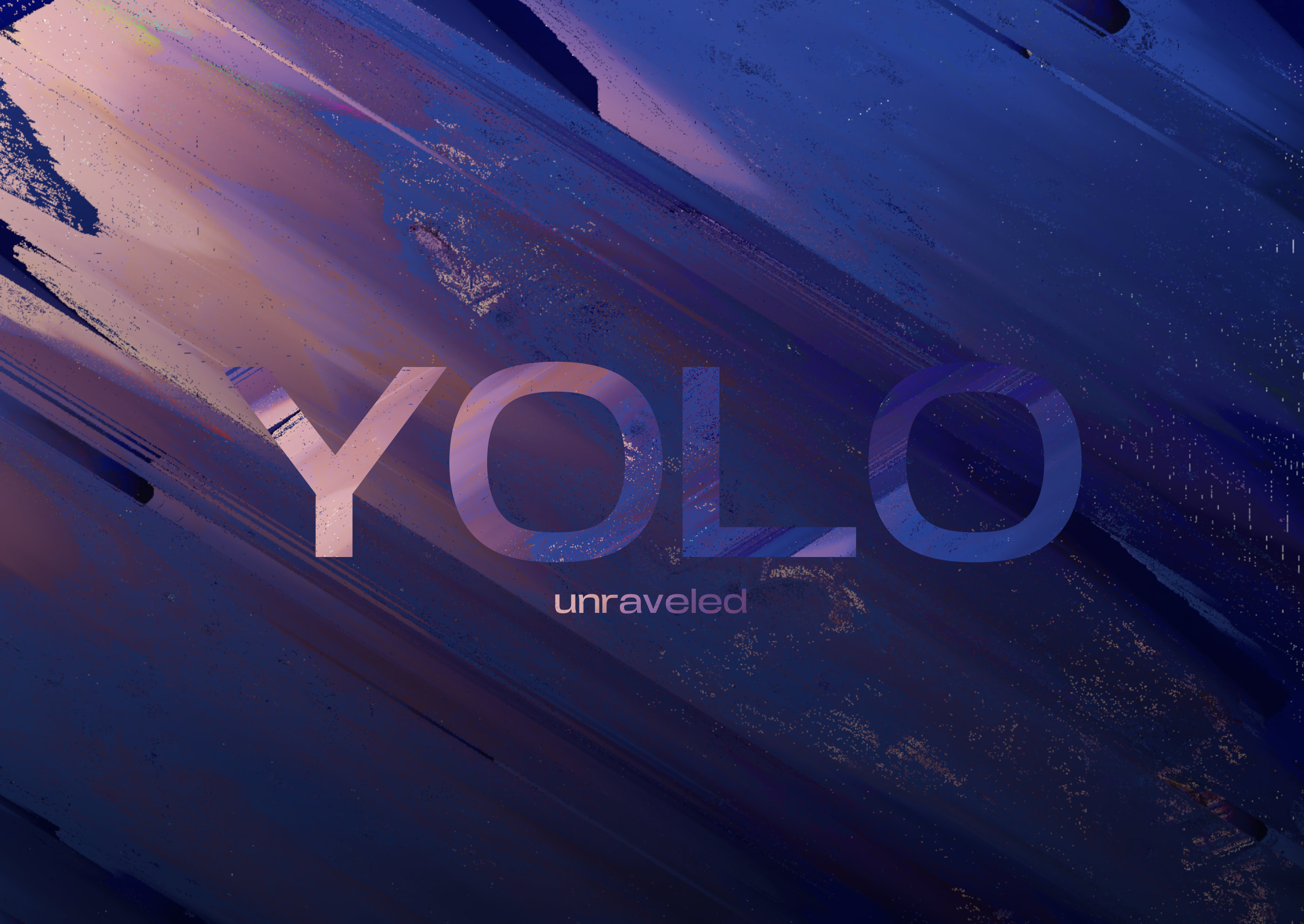
January 25, 2024
YOLO Unraveled: A Clear Guide
This comprehensive guide offers insights into the latest YOLO models and algorithms comparison, helping developers and researchers choose the most effective solution for their projects.
READ MORE
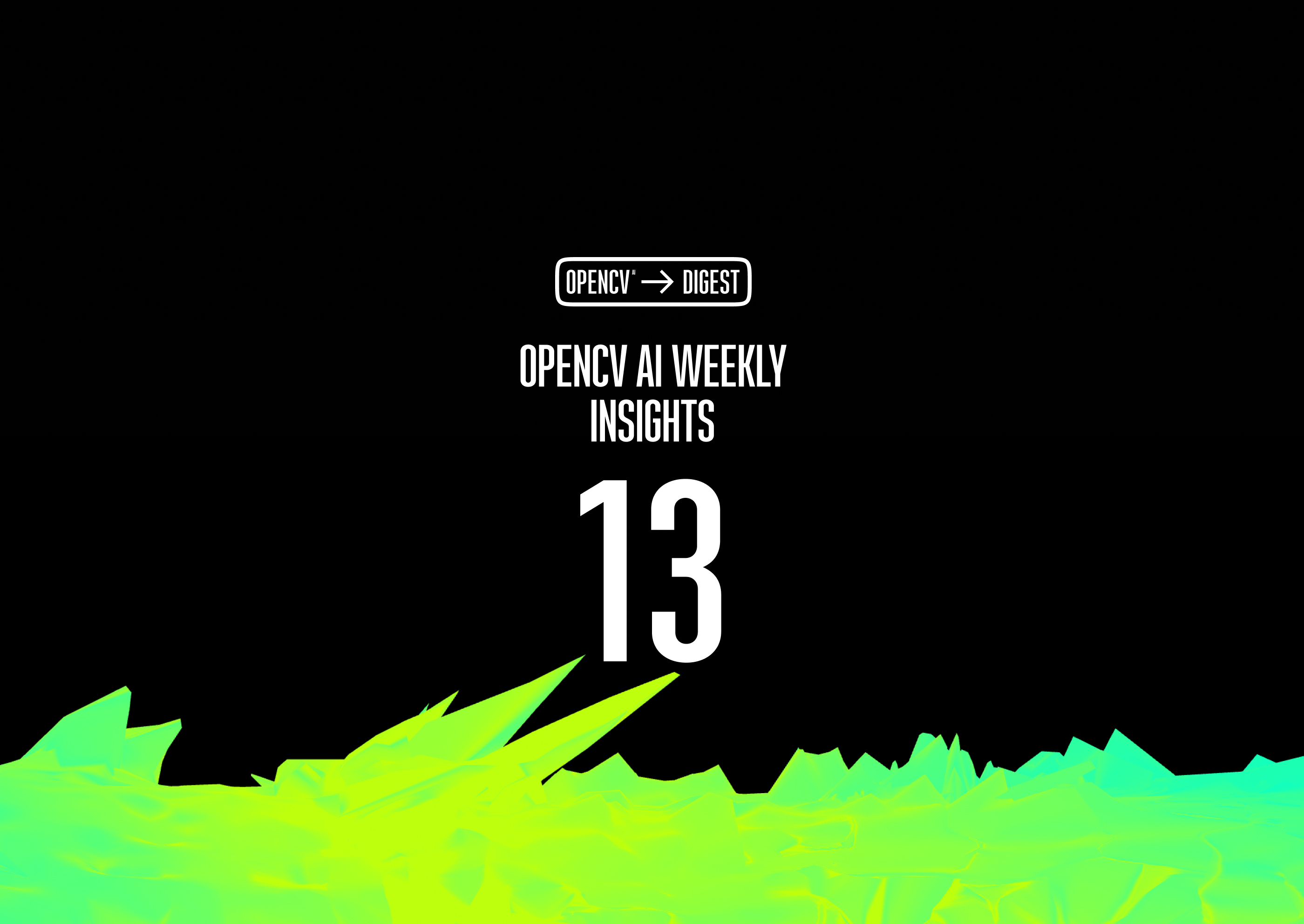
January 16, 2024
Digest 13 | OpenCV AI Weekly Insights
This week's digest covers the Rabbit R1 AI gadget for voice control, OpenAI's GPT Store for custom bot sharing, Anthropic's study on AI deception, and new AI e-commerce services from Google and Microsoft. These stories highlight key developments and concerns in the evolving field of AI.
READ MORE
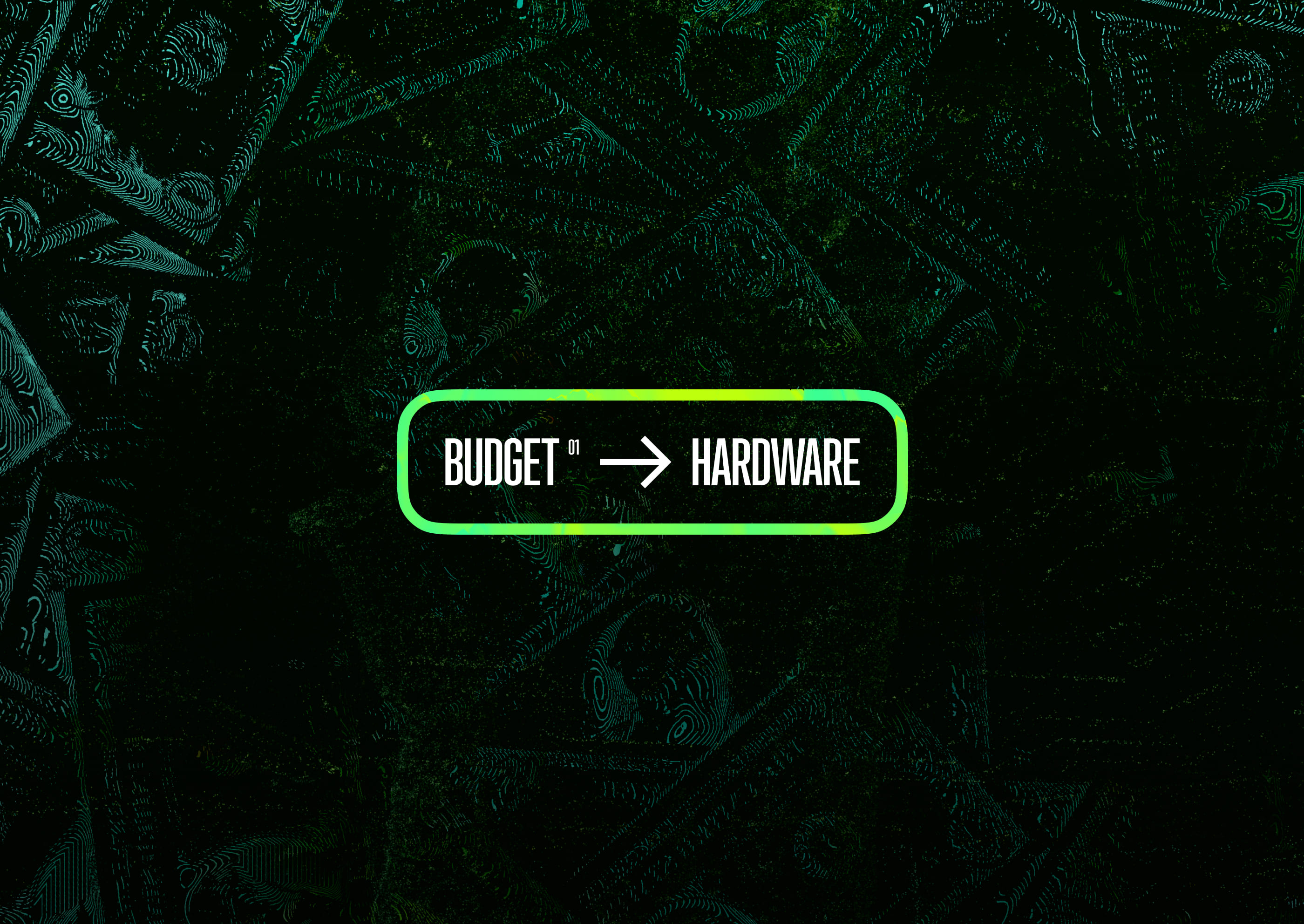
January 11, 2024
Practical guides to budget your AI and Computer Vision Solution
In 2024, as more companies integrate AI, many business owners face challenges. Discover the essential considerations for integrating AI into your business with OpenCV.ai's expert insights. From choosing the right camera for computer vision solutions to navigating diverse computing platforms, this article provides practical guidance. Explore the nuances of network and power optimization, and take the first step toward AI-driven success.
READ MORE

December 19, 2023
Computer Vision In Self-Driving Cars
The article explains the technology behind self-driving cars, focusing on computer vision and machine learning. It discusses how cars use cameras, LIDAR, and algorithms like YOLO and Deep SORT for detecting and tracking objects. The article also covers challenges and future trends in autonomous vehicle technology, including safety, public trust, and smart city integration.
READ MORE
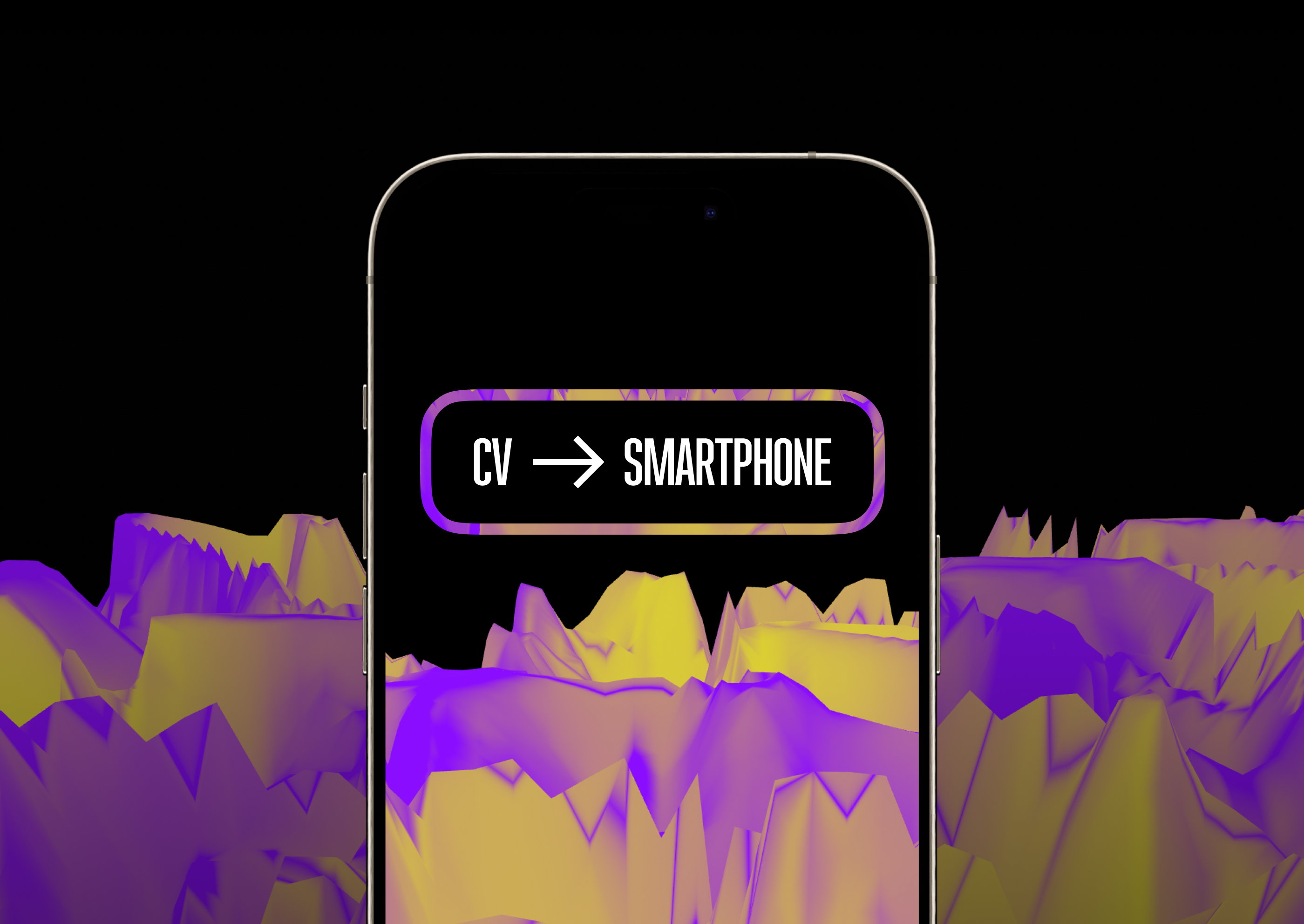
December 6, 2023
Computer Vision Applications In Your Smartphone
Discover how your smartphone is more than just a communication tool – it's a gateway to cutting-edge computer vision technology, transforming every interaction and enhancing security. It covers facial recognition for unlocking phones, augmented reality filters in social media apps, text recognition in images, and organizing photos by recognizing faces. These technologies improve phone security and user experience.
READ MORE
.jpg)
November 28, 2023
Digest 12 | OpenCV AI Weekly Insights
This week's OpenCV AI Weekly Insights Digest covers key AI developments, including Stability AI's new Stable Video Diffusion model for video generation, Tesla's Full Self-Driving v12 update testing, a U.S. investigation into UAE's AI firm G42 for data risks linked to China, and a study showcasing AI's advancements in microscopy image analysis.
READ MORE
.jpg)
November 22, 2023
Digest 11 | OpenCV AI Weekly Insights
This week's OpenCV AI Weekly Insights Digest covers major developments in AI, including DeepMind's new music generation model Lyria, NVIDIA's H200 GPU enhancing AI performance, Meta's innovative video and image editing tools, and significant leadership changes at OpenAI and Microsoft.
READ MORE
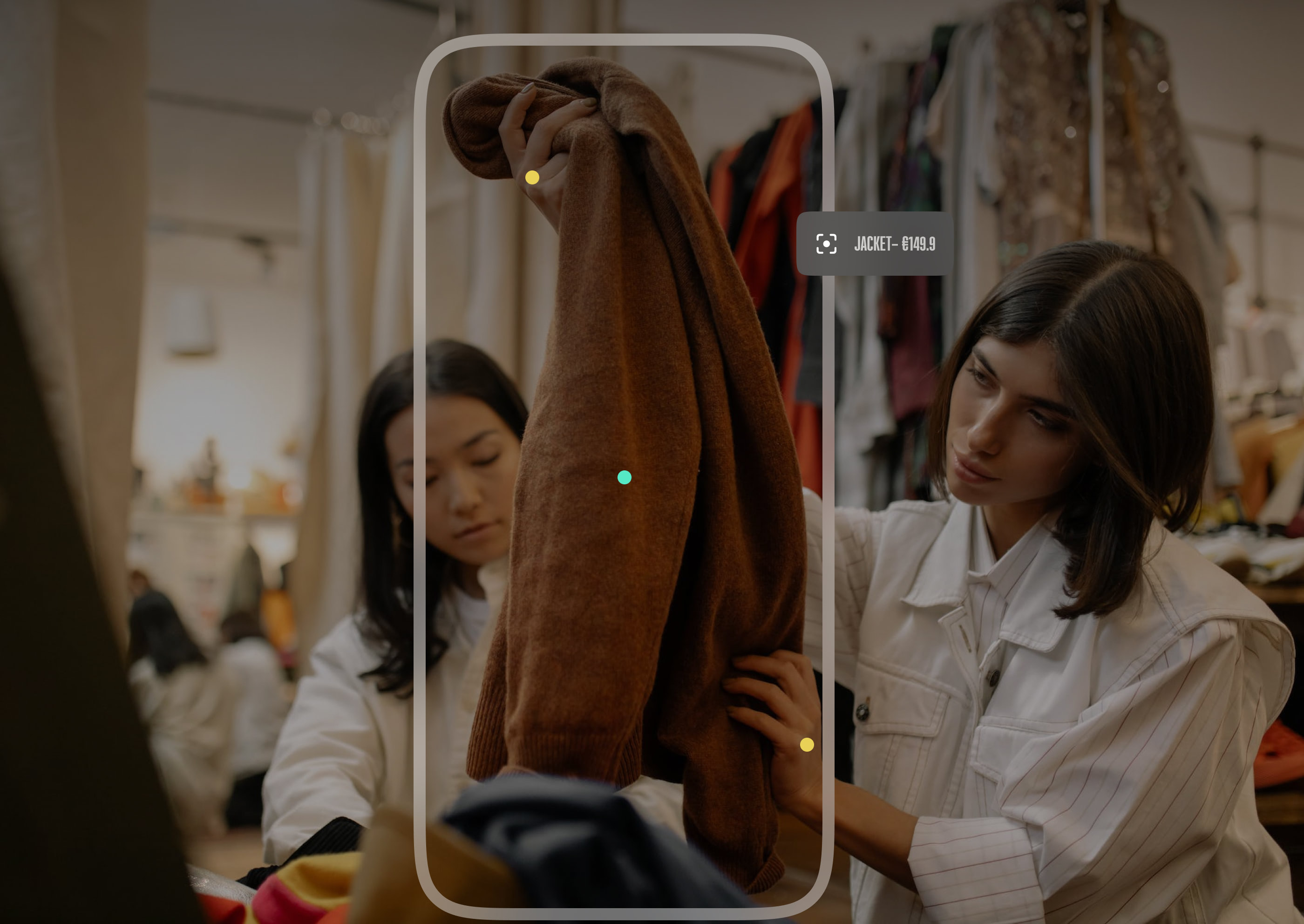
November 13, 2023
Computer Vision in Retail: Cashier-less Stores
The retail sector is evolving with the help of artificial intelligence and computer vision, leading to the development of stores without cashiers. This technology helps in managing inventory and tracking stock levels automatically. It also analyzes customer behavior in stores, in order to improve store arrangement and marketing, making shopping easier and more tailored to individual preferences.
READ MORE
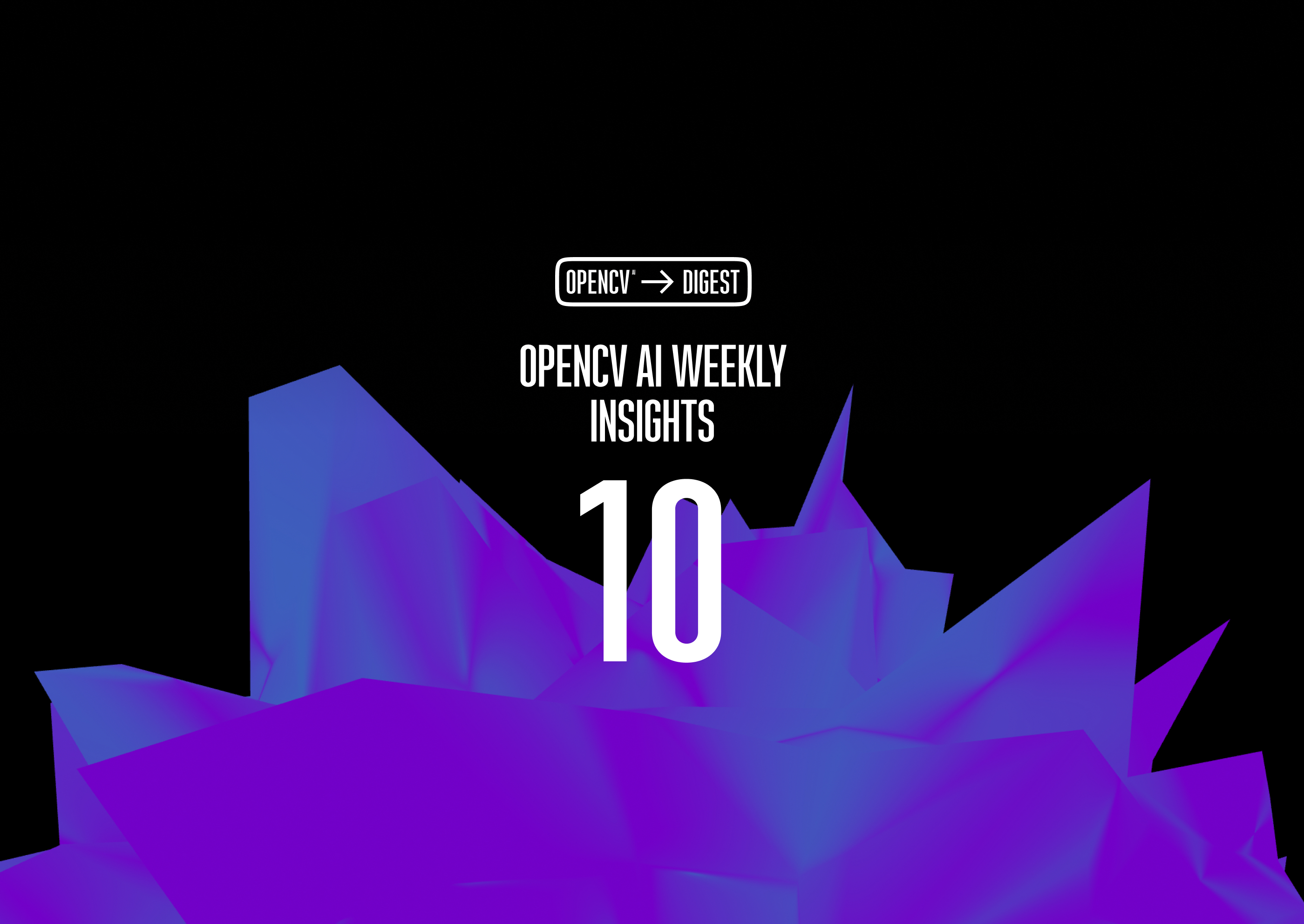
November 7, 2023
Digest 10 | OpenCV AI Weekly Insights
In this week's AI update, we consider the following news: the historic AI summit, where over 25 countries unite to collectively govern AI risks; Elon Musk introduces Grok, an AI chatbot; Apple's plans for the 2024 Apple Watch with new health features; and The Beatles' surprise release of "Now and Then" using AI technology.
READ MORE
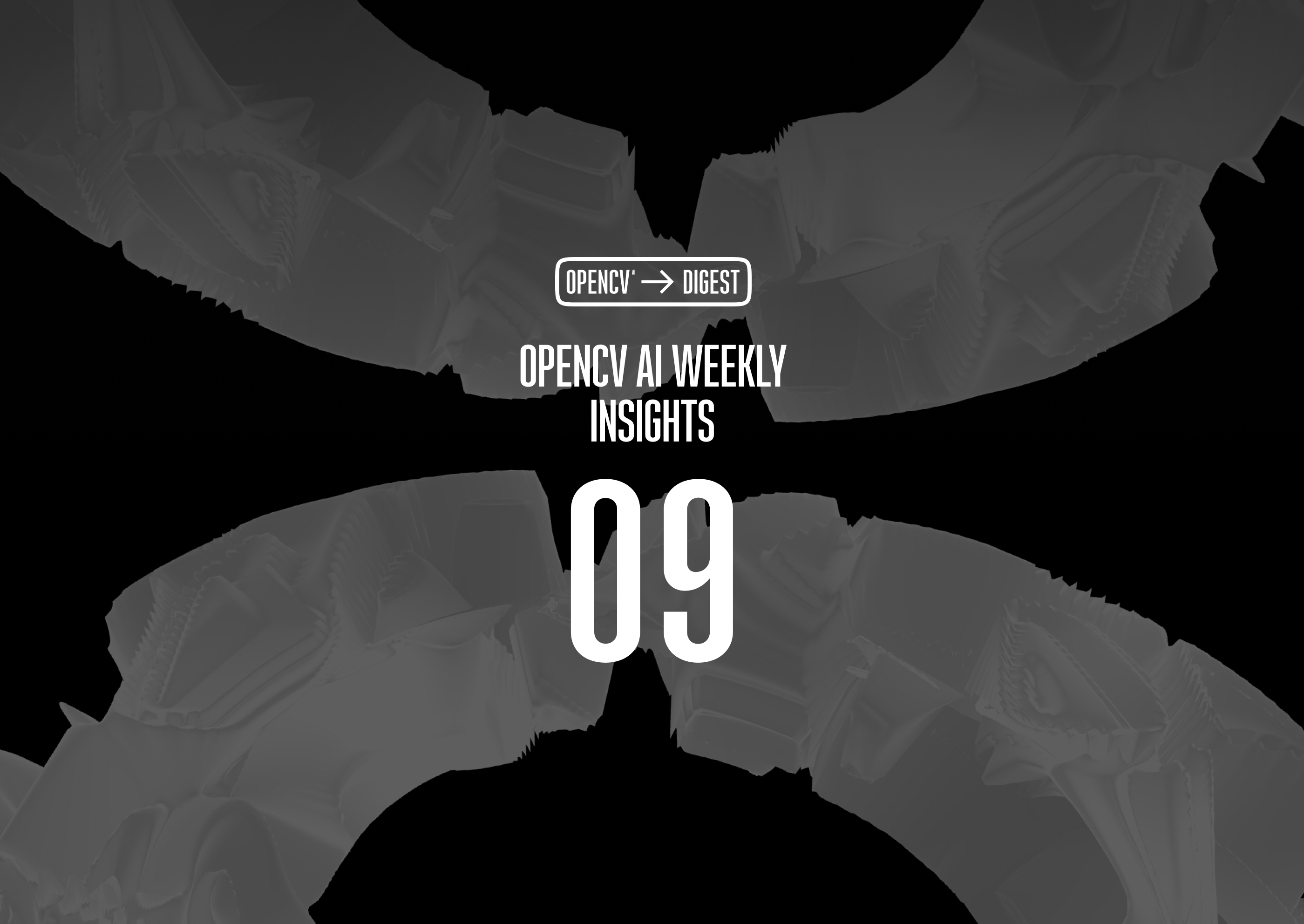
October 30, 2023
Digest 9 | OpenCV AI Weekly Insights
In this week's AI update, we bring you the latest developments in the tech world: Boston Dynamics' integration of OpenAI's GPT-4 into Spot, the robot dog, enhancing its conversational capabilities; Amazon's beta testing of AI image generation tools for advertisers; Google's $2 billion investment in Anthropic, and more.
READ MORE
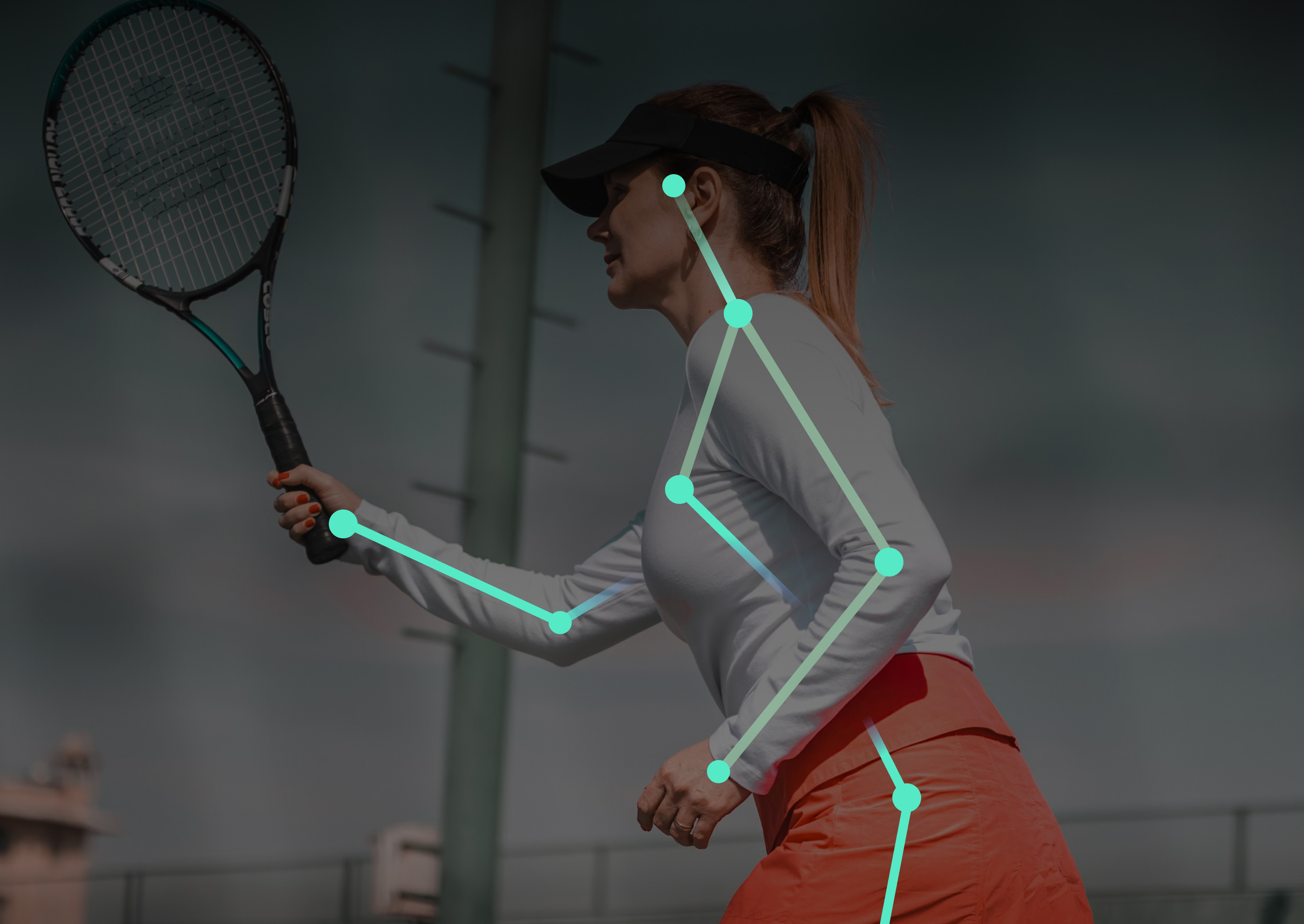
April 10, 2024
AI and Fitness: Explore Exercise with Pose Tracking Technology
Meet AI and fitness combination: pose tracking technology analyzes and improves exercise routines in real-time.
READ MORE
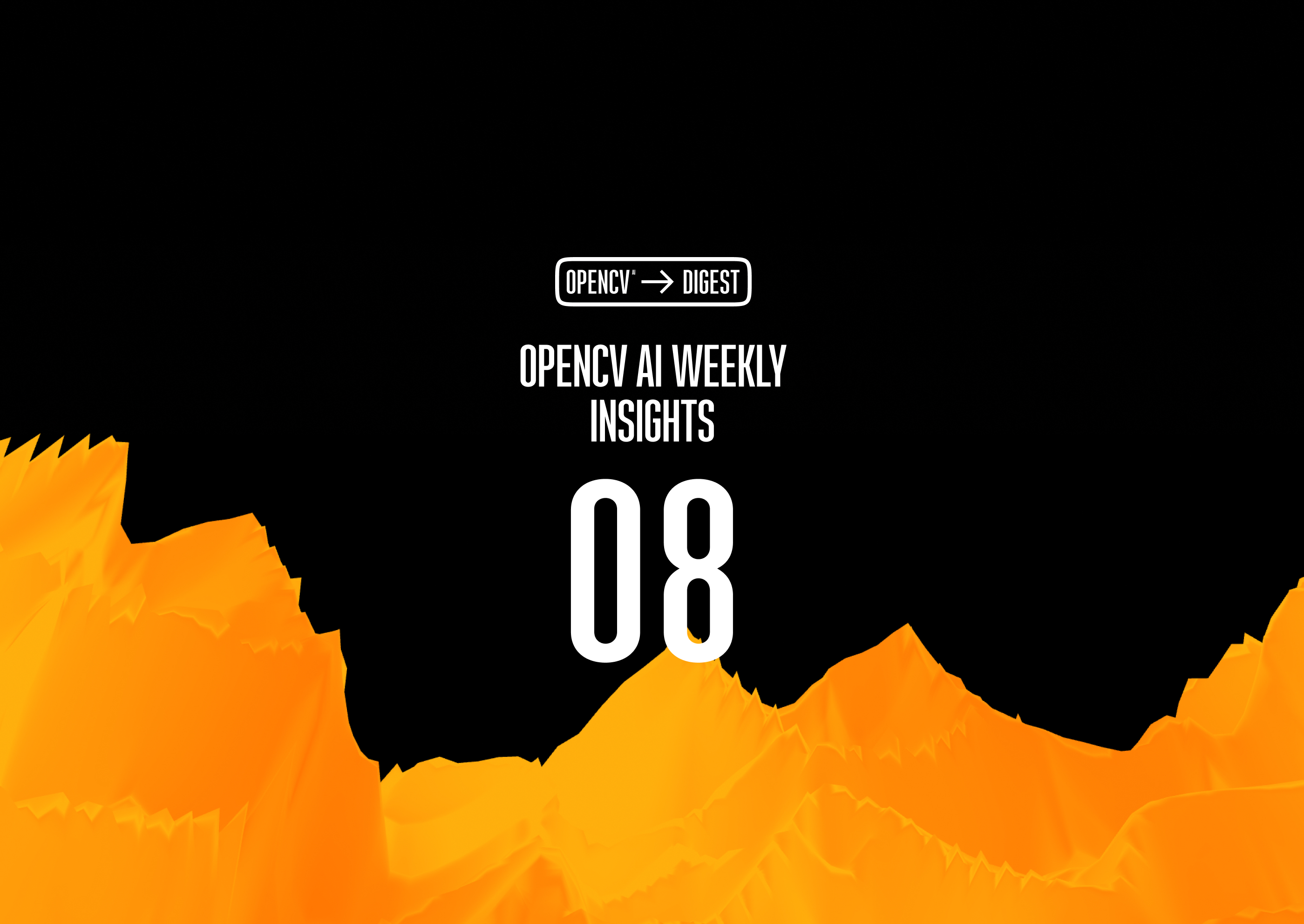
October 25, 2023
Digest 8 | OpenCV AI Weekly Insights
Here you will find a recap of last week and what's happened in the digital AI world: Highly-skilled robots by Nvidia, Apple plans to incorporate AI into their products, a comprehensive study about LLMs bias in medicine, and more.
READ MORE

October 19, 2023
Computer Vision in Agriculture. Challenges & Solutions
Computer vision is helping the agriculture industry with close monitoring of crops and soil, early detection of diseases, forecasting and even livestock management, improving efficiency and sustainability. Explore the use of synthetic data in agriculture to enhance real-world datasets, making computer vision models more robust and adaptable to various conditions like diverse weather scenarios and lighting conditions.
READ MORE
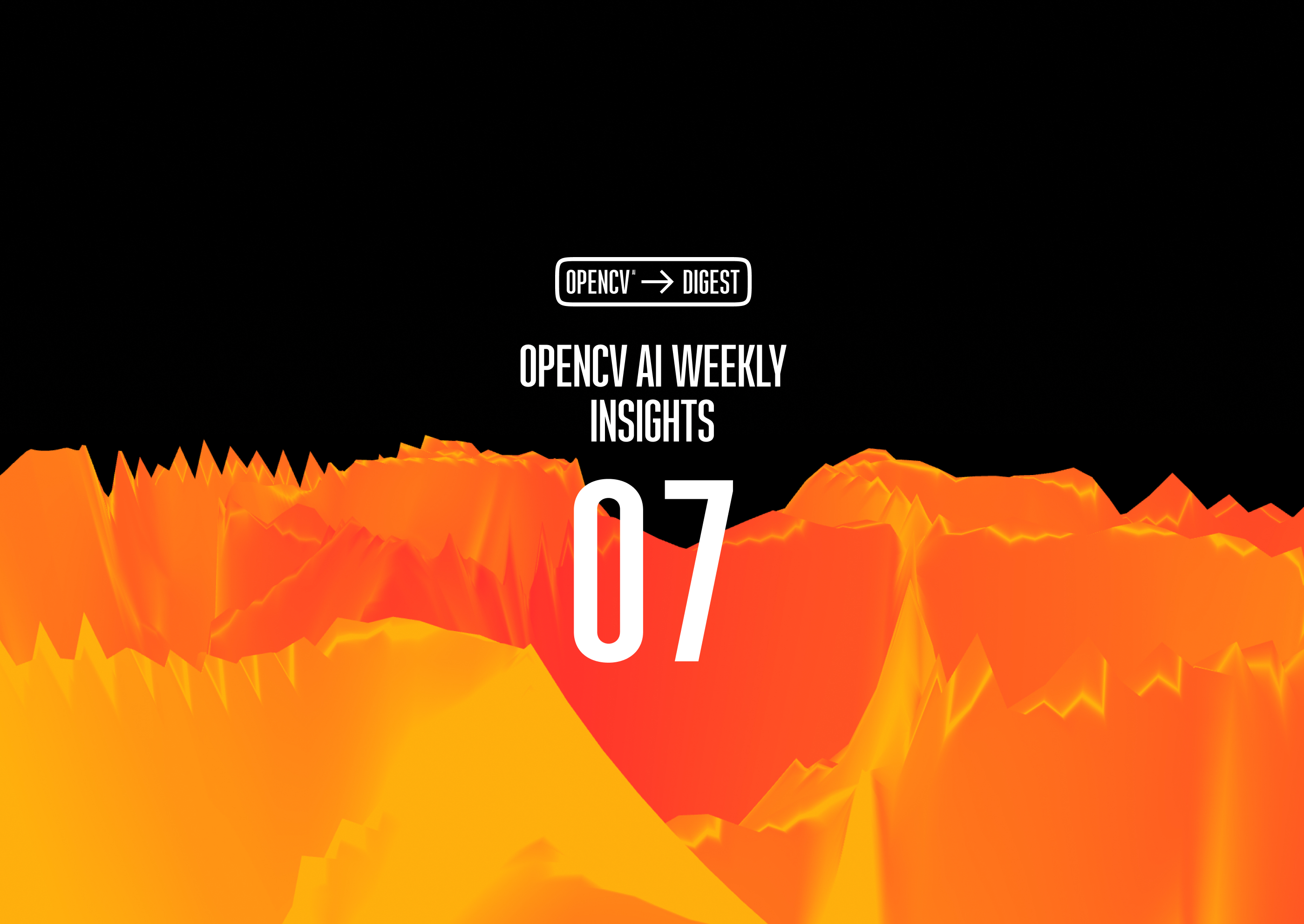
October 17, 2023
Digest 7 | OpenCV AI Weekly Insights
Check the latest news in the AI field: from Google Search image generation feature to AI celebrity avatars, which replace traditional influencers!
READ MORE
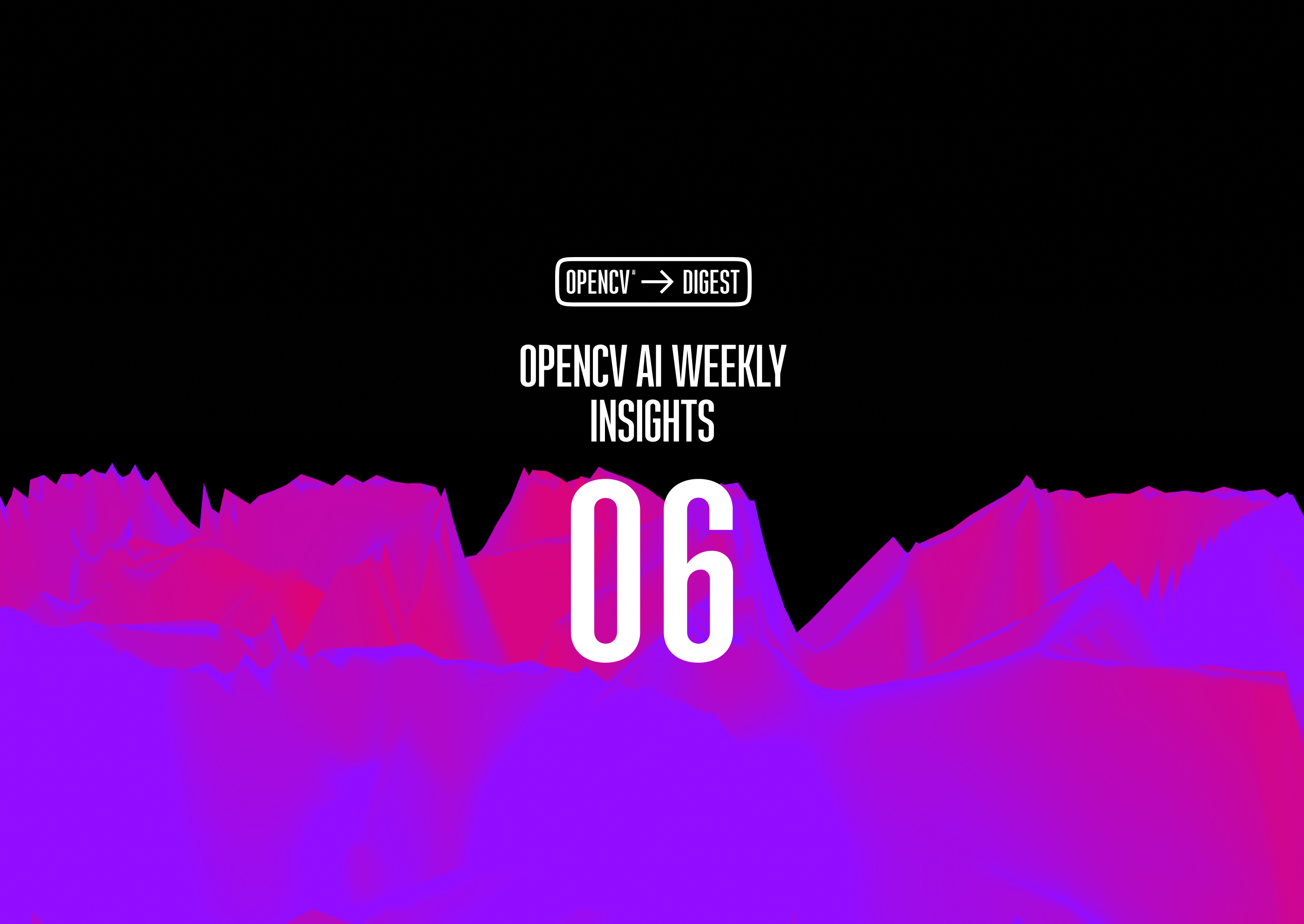
October 10, 2023
Digest 6 | OpenCV AI Weekly Insights
Here's our weekly roundup of newsworthy developments from the world of computer vision and AI.
READ MORE
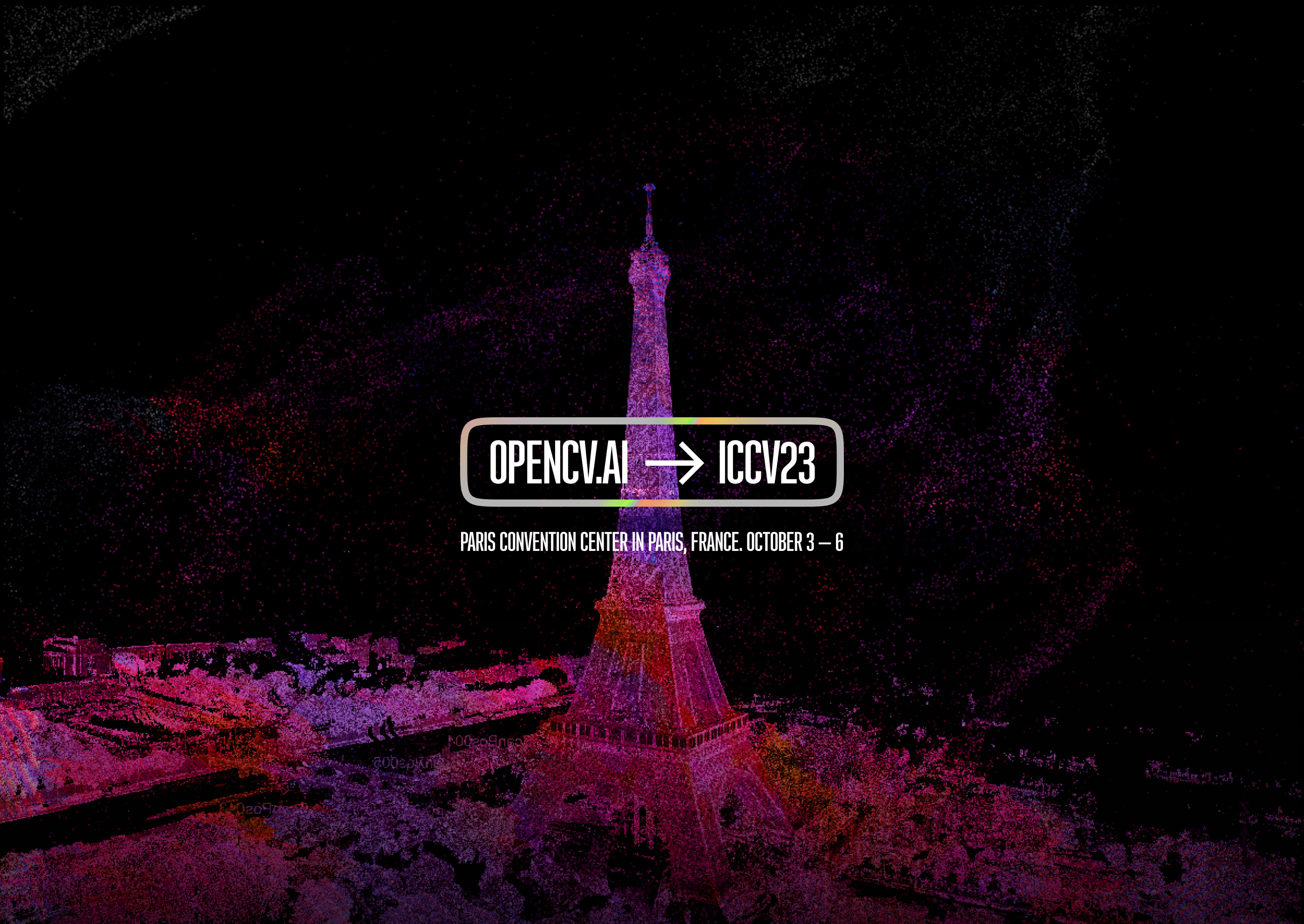
September 29, 2023
The International Conference on Computer Vision
In this special blog article, we will document our visit to the ICCV convention.
READ MORE
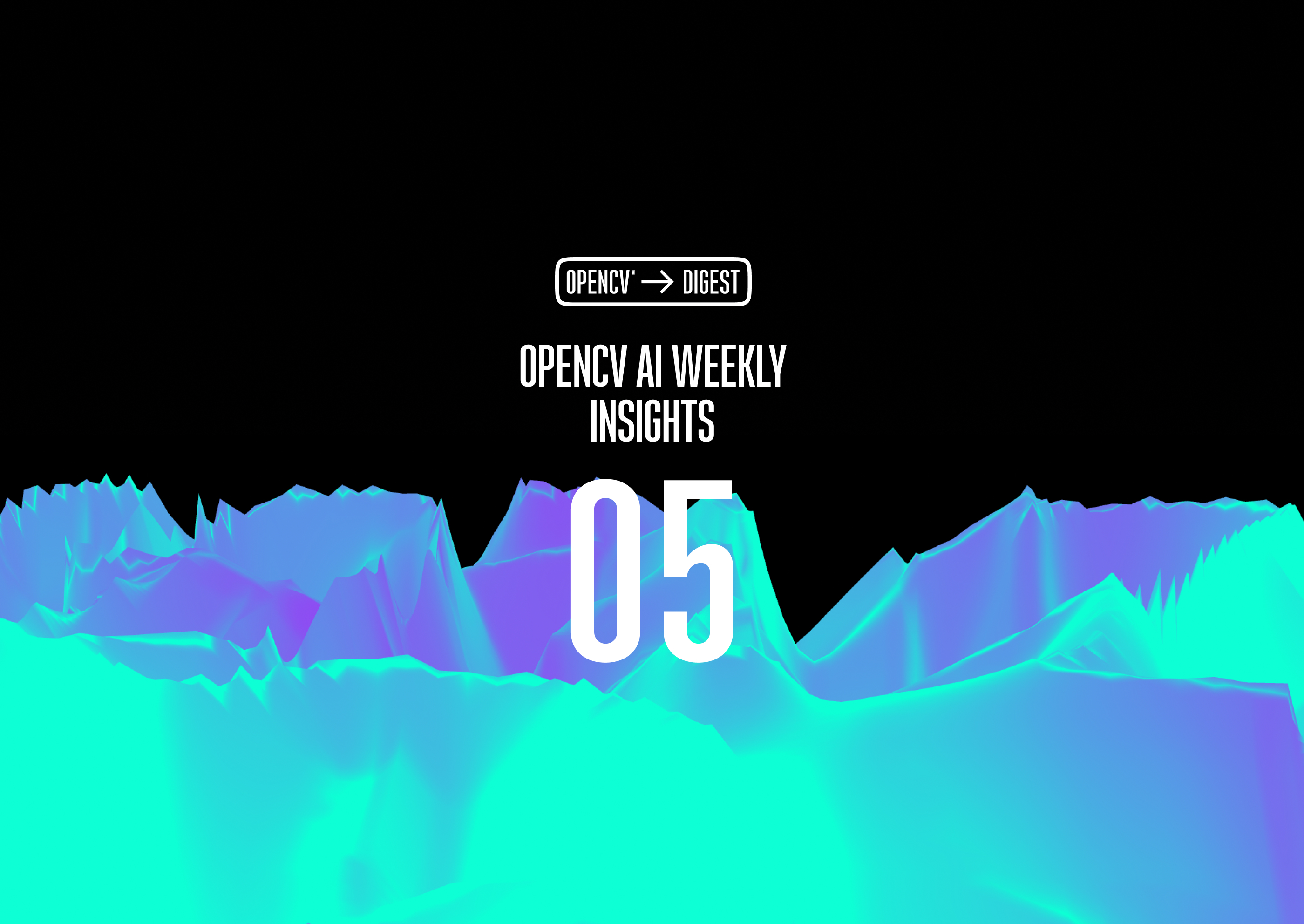
September 12, 2023
Digest 5 | OpenCV AI Weekly Insights
Here's our weekly roundup of newsworthy developments from the world of computer vision and AI.
READ MORE
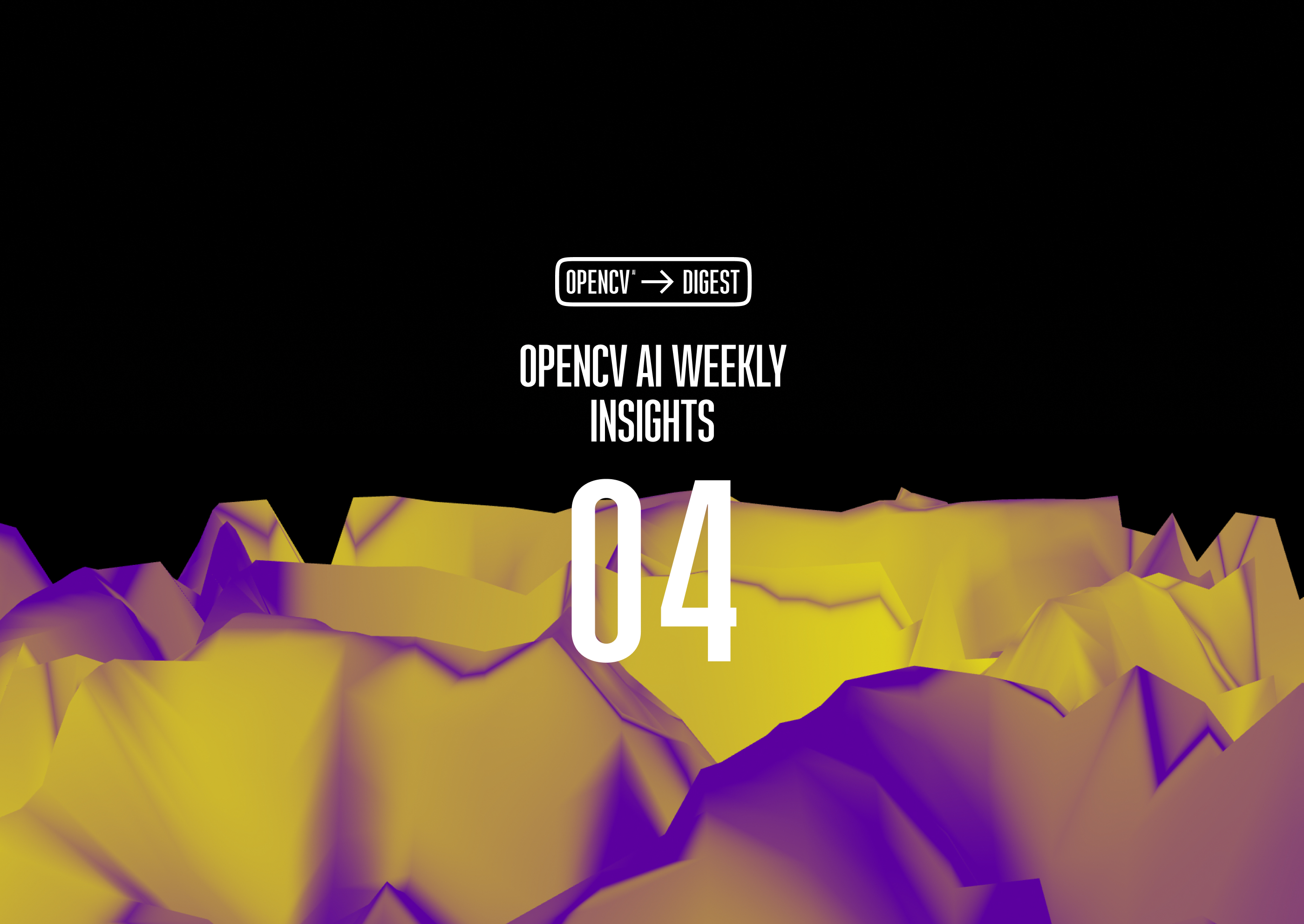
September 5, 2023
Digest 4 | OpenCV AI Weekly Insights
What happened in the AI world this week? Let's find out! From groundbreaking headlines to jaw-dropping breakthroughs, we've got the scoop.
READ MORE
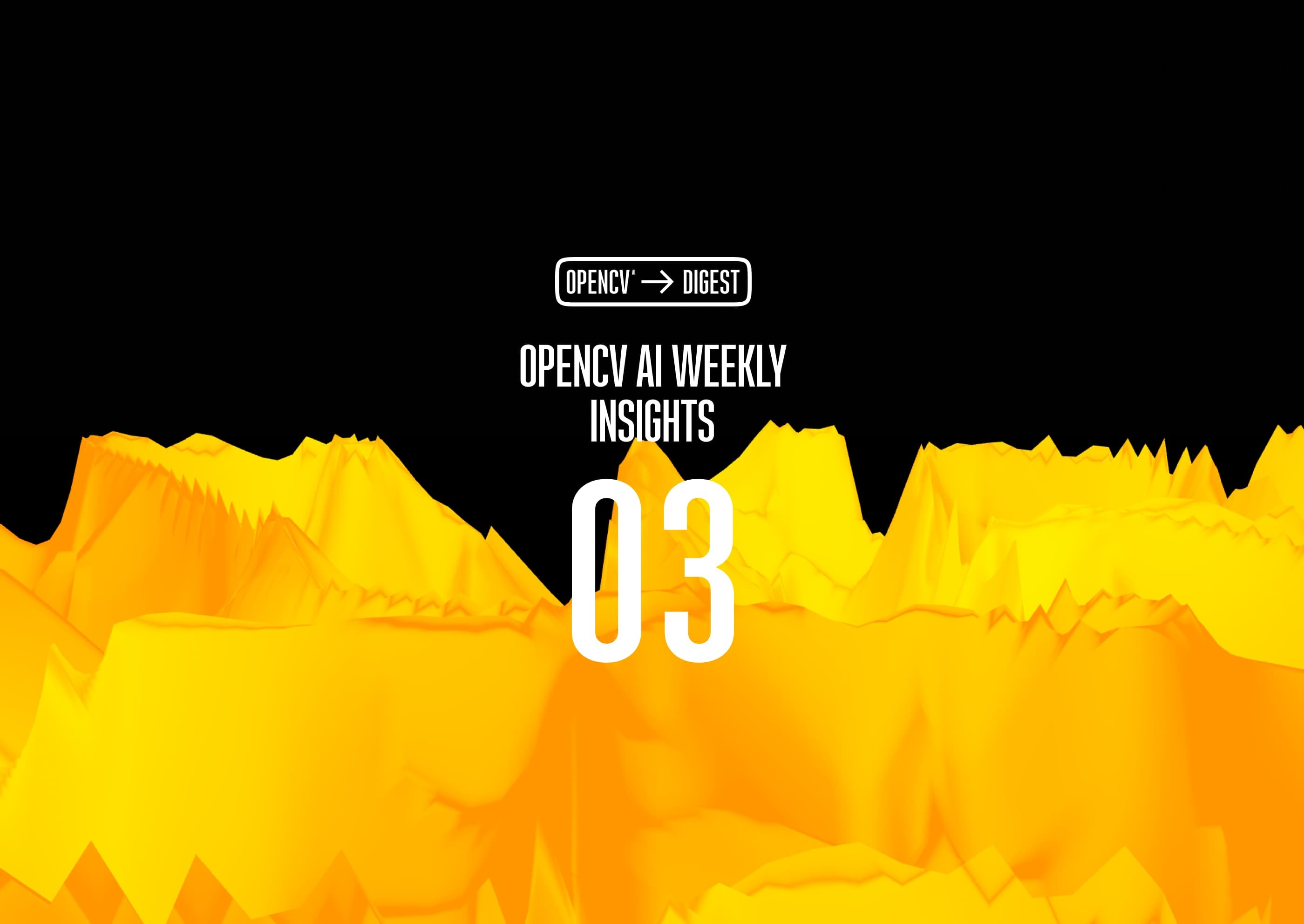
August 29, 2023
Digest 3 | OpenCV AI Weekly Insights
What happened in the AI world this week? Let's find out! ChatGPT Fine-Tuning, Facebook released AI tool for coding, and more!
READ MORE
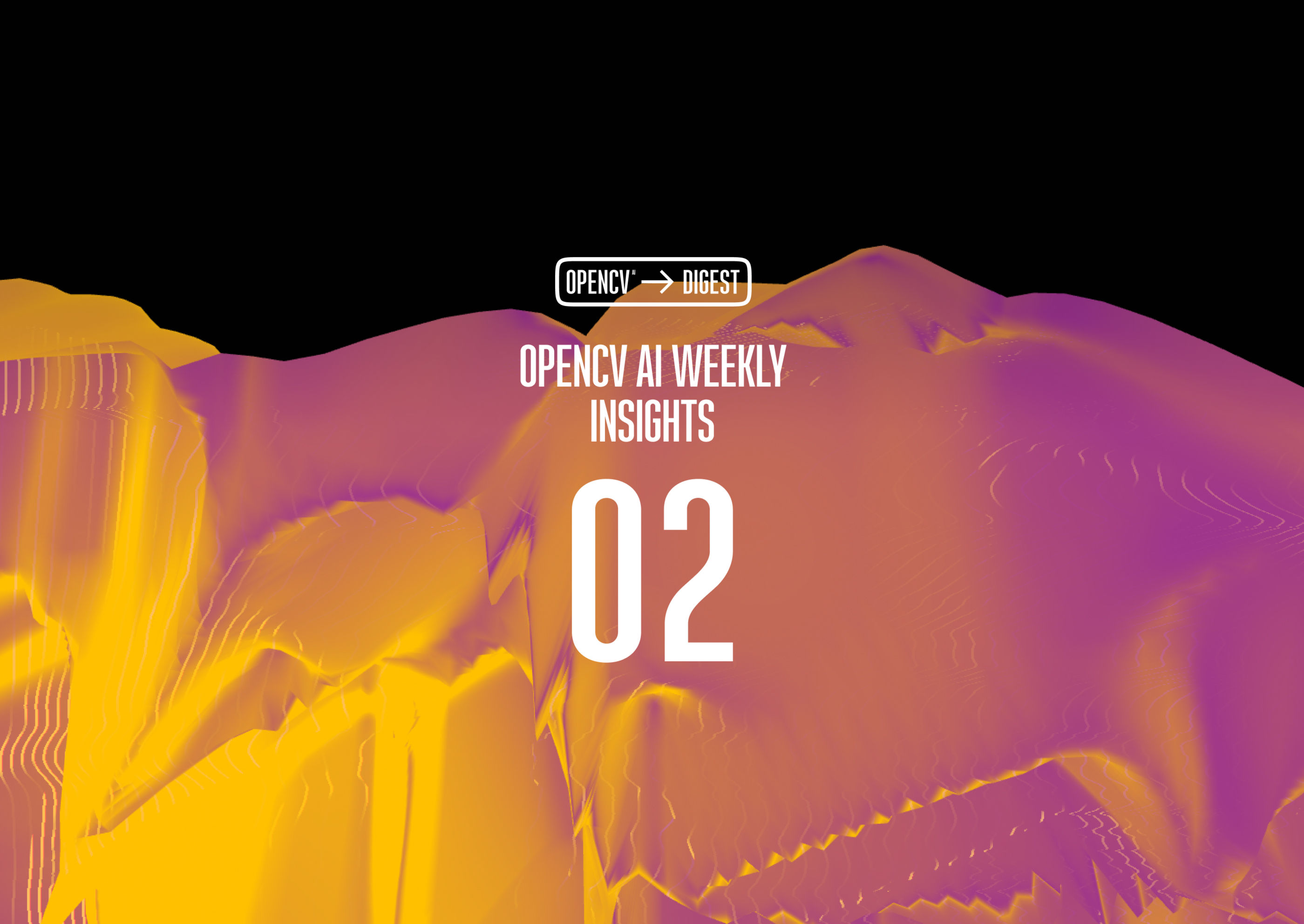
August 21, 2023
Digest 2 | OpenCV AI Weekly Insights
What happened in the AI world this week? Let's find out! NVIDIA's Open-Source 3D Tool Release, San Francisco's Autonomous Driving Pause, and more!
READ MORE
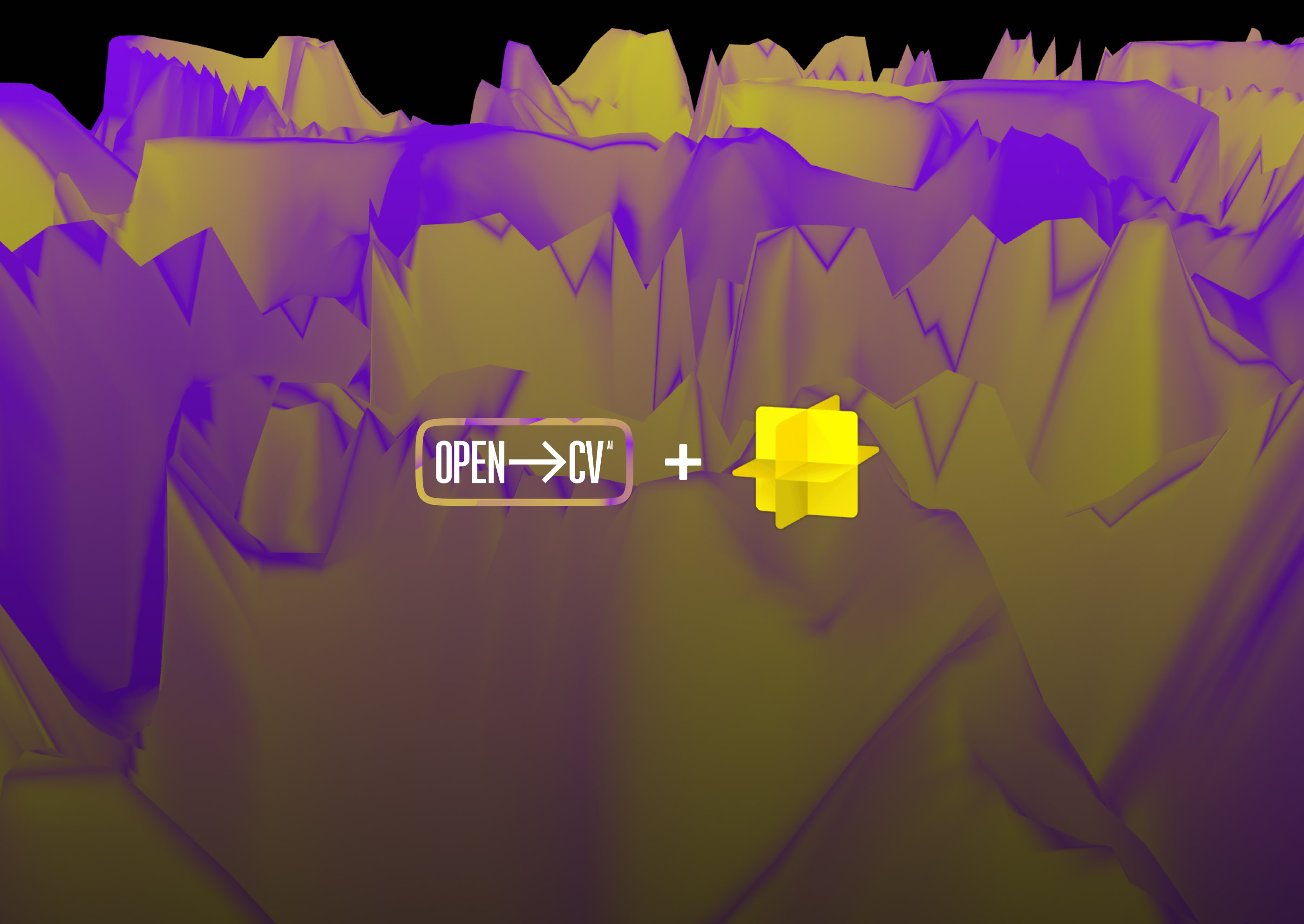
August 16, 2023
Snapchat Lens for Optical Character Recognition by OpenCV.ai
Join us in a reveal of our collaboration with Snap, to create a real-time and accurate Text Recognition tool!
READ MORE
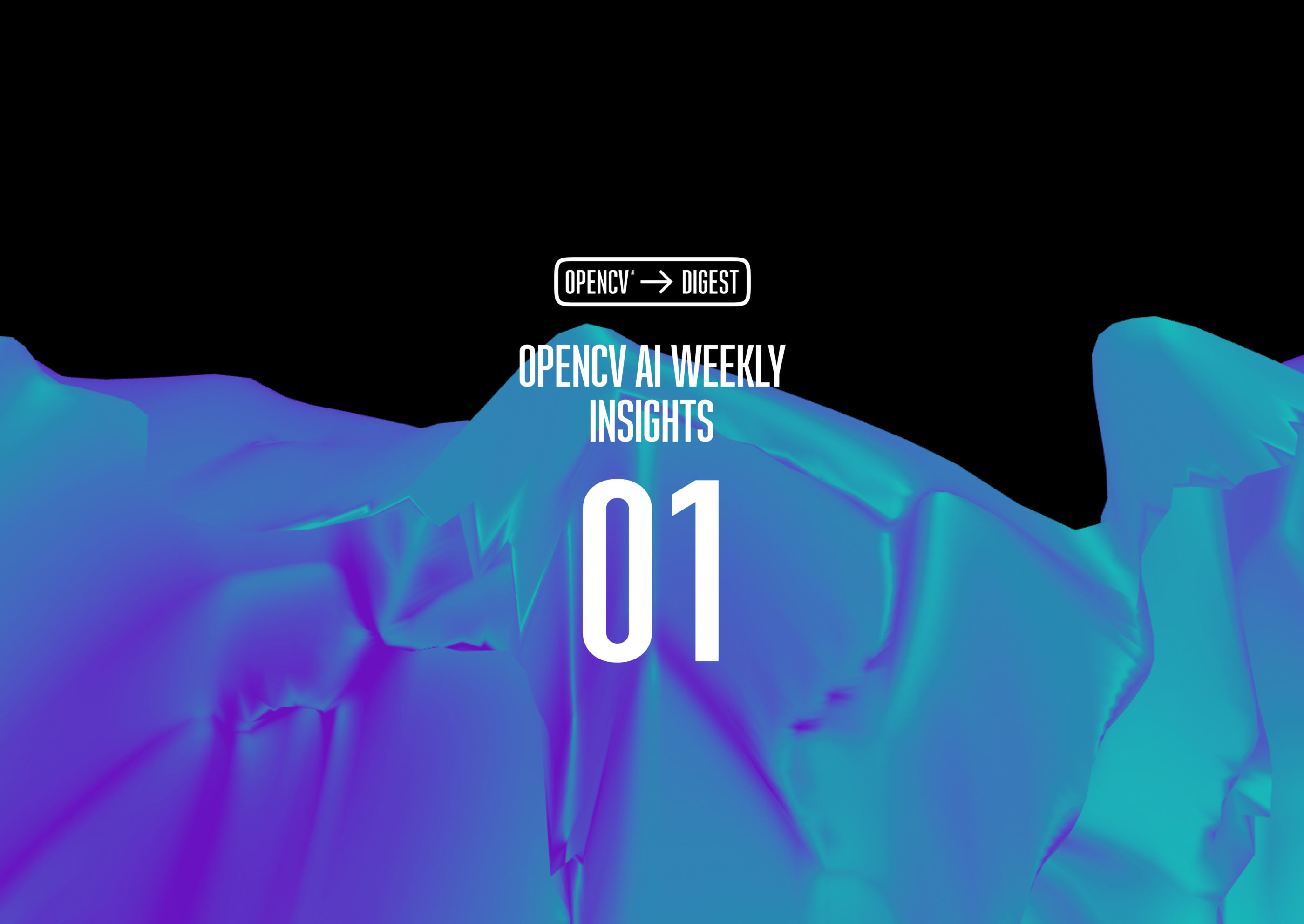
August 14, 2023
Digest 1 | OpenCV AI Weekly Insights
What happened in the AI world this week? Let's find out! From groundbreaking headlines to jaw-dropping breakthroughs, we've got the scoop.
READ MORE
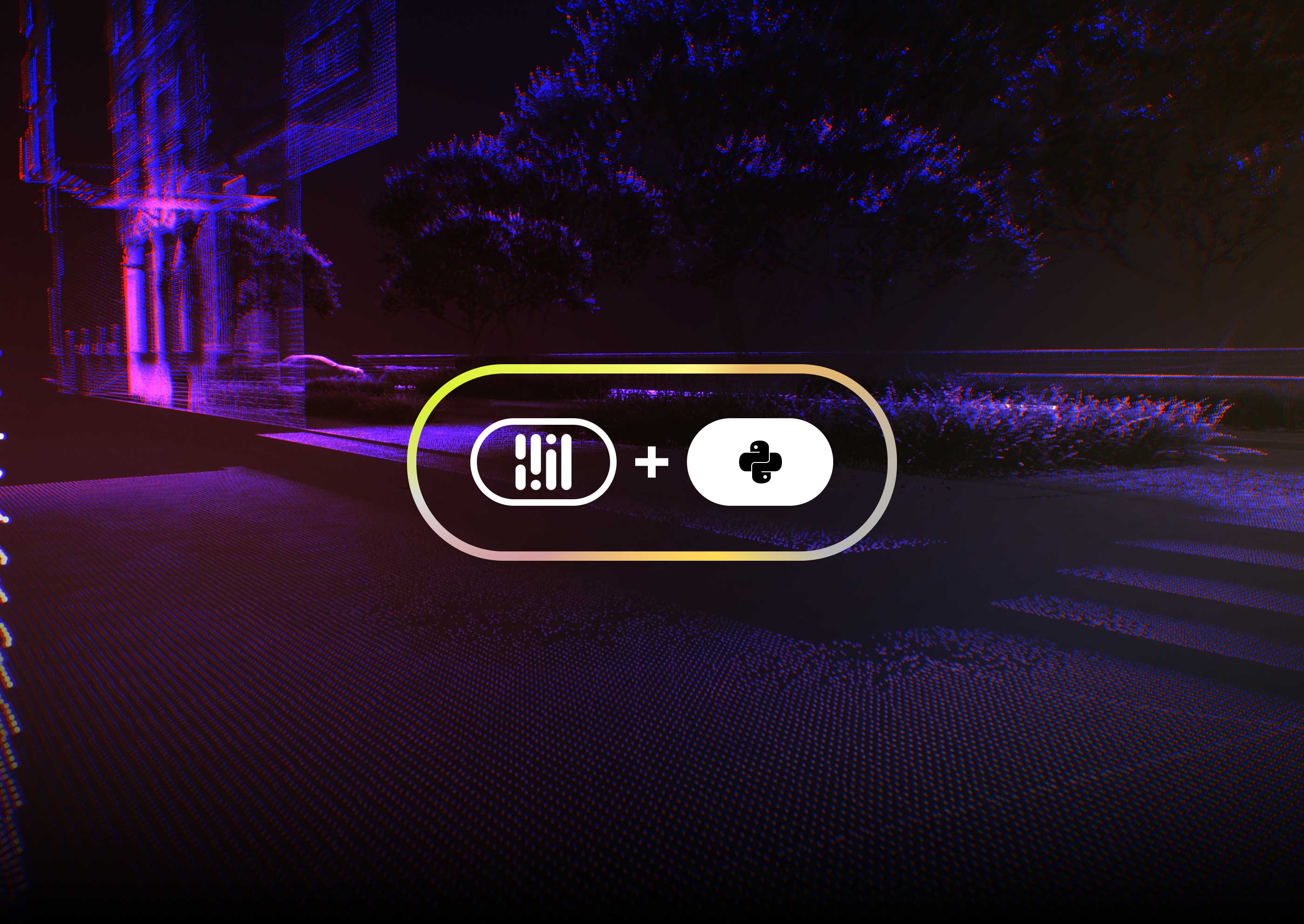
April 10, 2024
Look into MediaPipe solutions with Python
Discover the future of media processing with MediaPipe. This open-source framework empowers you to harness machine learning for video and audio, operating in real time on diverse devices.
READ MORE
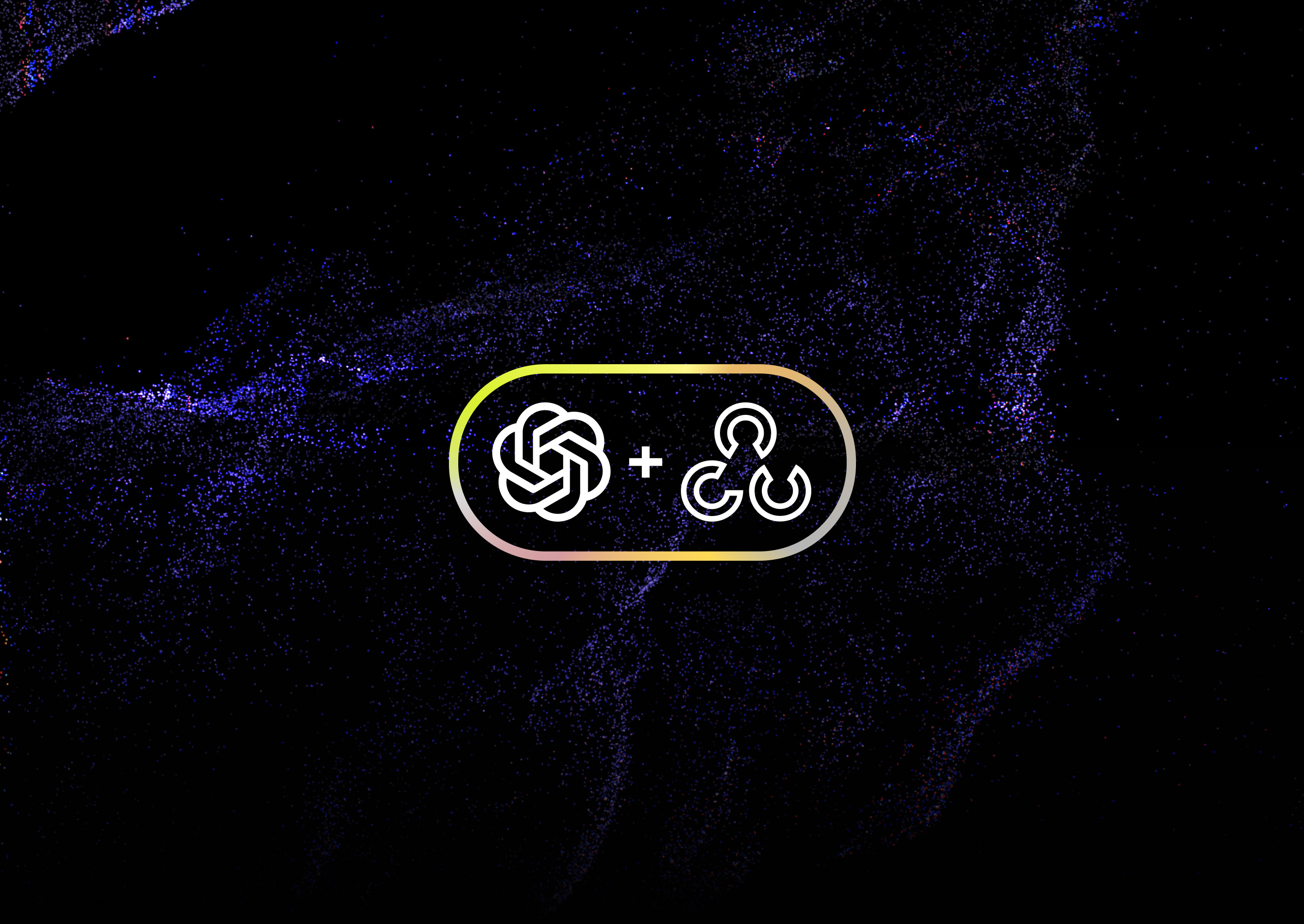
April 10, 2024
Getting the Hang of OpenCV’s Inner Workings with ChatGPT
Keeping pace with the evolution of technology and utilizing it judiciously, this blog explores how ChatGPT can serve for code development debugging.
READ MORE
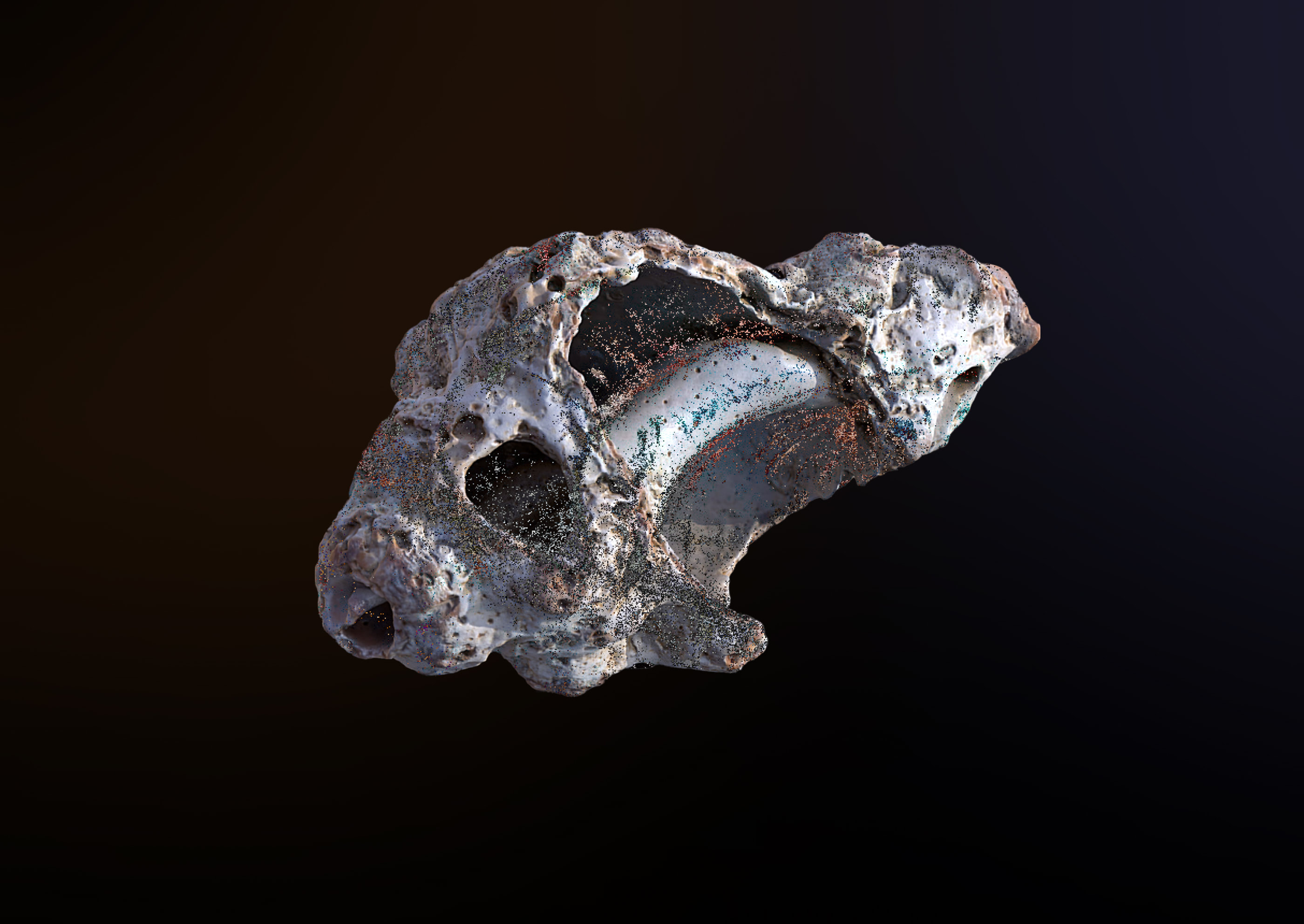
July 17, 2023
Tech track #4. NeRF: Photorealistic Image Synthesis
NeRF is an innovative technology that generates photorealistic images of scenes from novel viewpoints using a neural network and volume rendering techniques. This article explores NeRF components, training, strengths and limitations, and advancements in modern NeRF-based solutions.
READ MORE
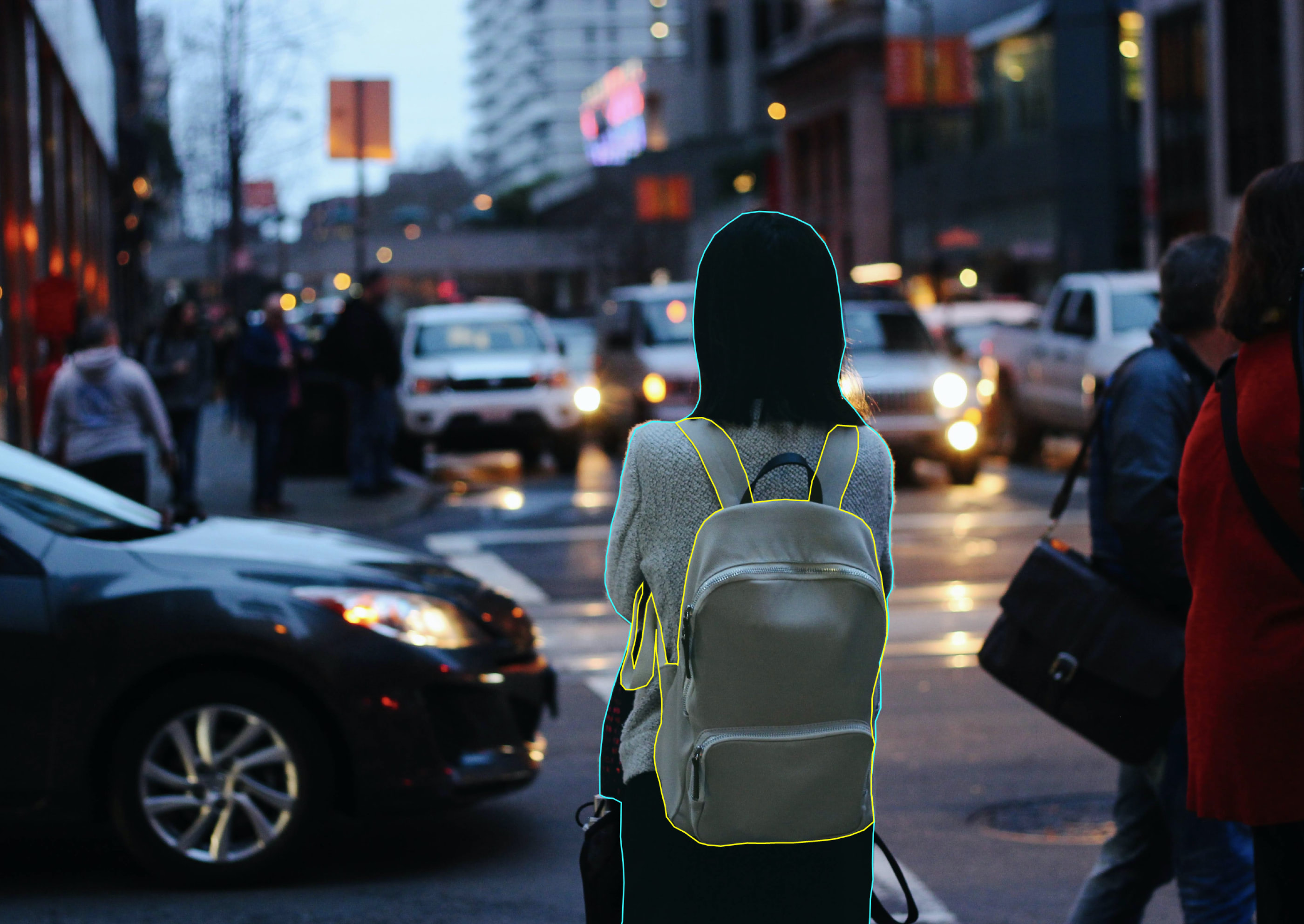
July 3, 2023
Tech track #2. Fast SAM review
Fast SAM, an innovative approach to the Segment Anything Task, drastically increases SAM model speed by 50 times. It introduces prompts to facilitate a broad range of problem-solving, breaking barriers of traditional supervised learning. This blog post will briefly explain the segment anything task and the Fast SAM approach.
READ MORE

April 10, 2024
Tech track #3. Object position in 3D space - 6Dof metrics overview
In the vast world of 3D object pose estimation, one group of tasks demands a distinct spotlight. This is where we delve into predicting the position of rigid objects in 3D.
READ MORE
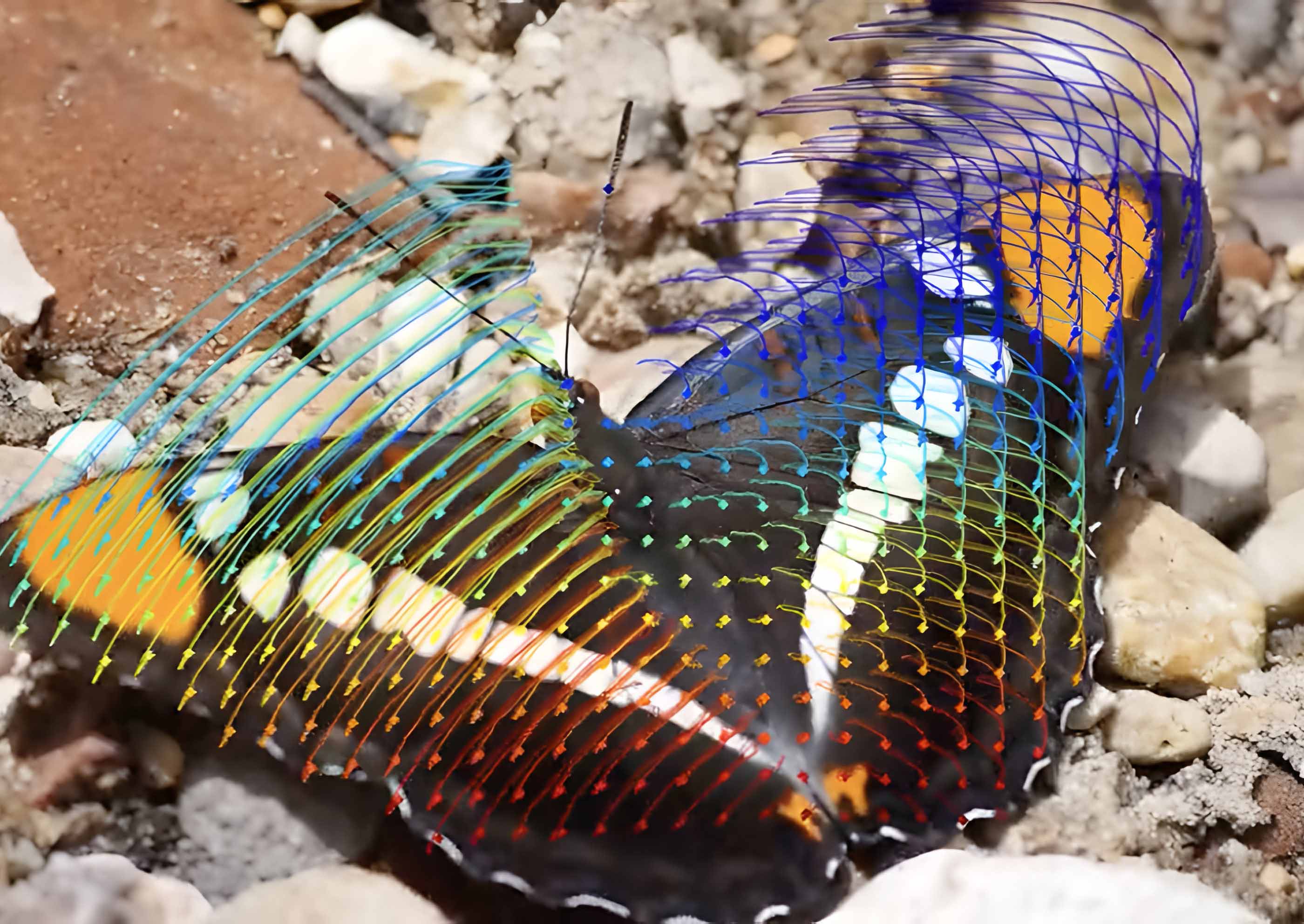
June 15, 2023
Tech track #1. "Tracking Everything Everywhere All at Once" review
The "Tracking Everything Everywhere All at Once" paper, a collaborative work by Cornell University, Google Research, and UC Berkeley, offers a breakthrough solution to the problem of tracking any point in video footage. The method maps each pixel from every frame into a common 3D space, tracing its trajectory across time. This method is not designed for real-time tracking but for in-depth recorded video analysis. In this article, we highlight the most exciting points of this paper.
READ MORE
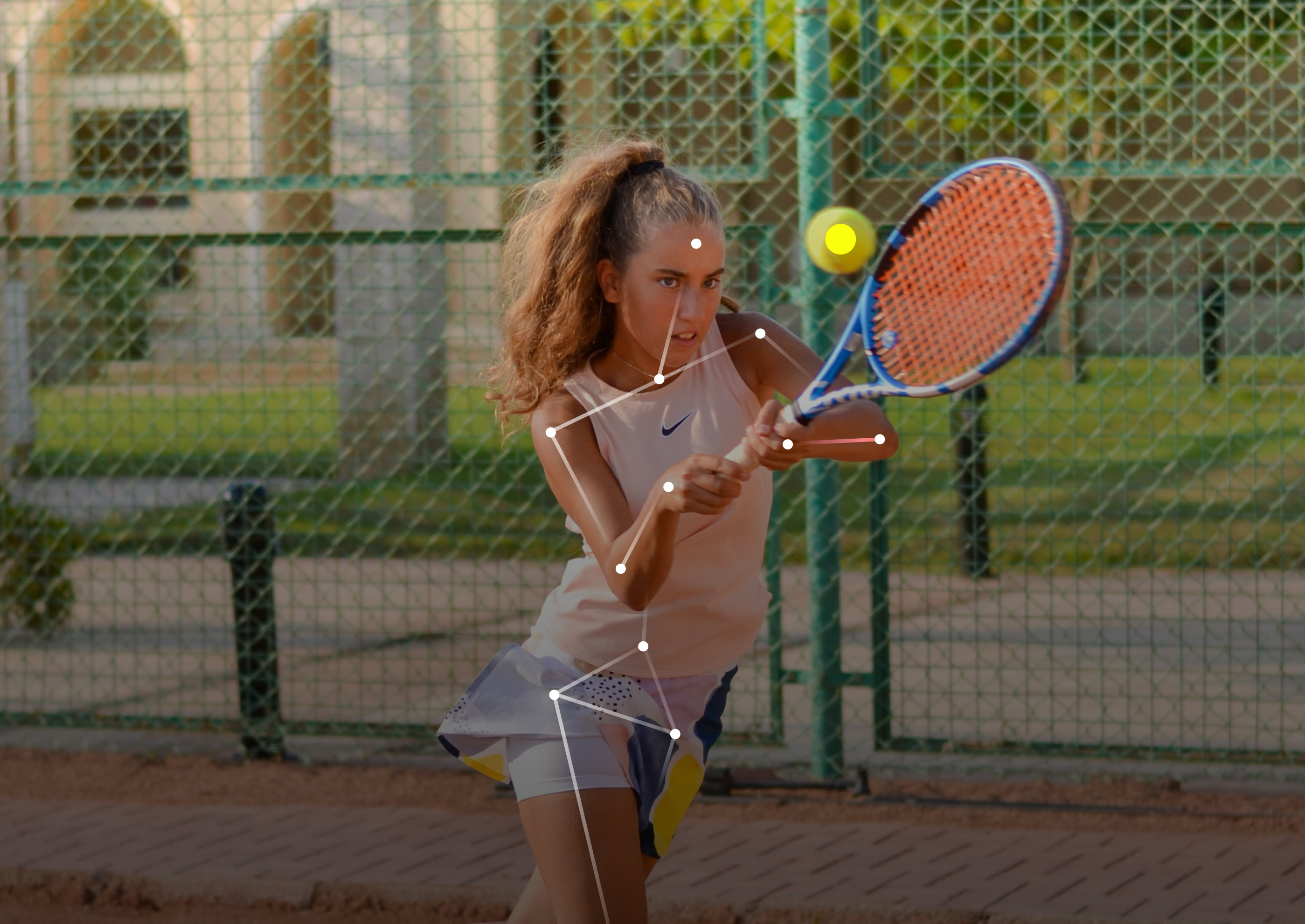
May 10, 2023
Applied AI #2. Revolutionizing Tennis with AI and Computer Vision
At OpenCV.ai, we have been developing cutting-edge AI-based analytic algorithms for tennis, aiming to reshape the way professional athletes train and perform in various sports. The growing influence of AI in the sports industry has led to significant advancements in training methods and analytics, providing invaluable insights for athletes and coaches alike. Here, we break down the key benefits and applications of AI and Computer Vision in tennis, showcasing our expertise in this domain.
READ MORE
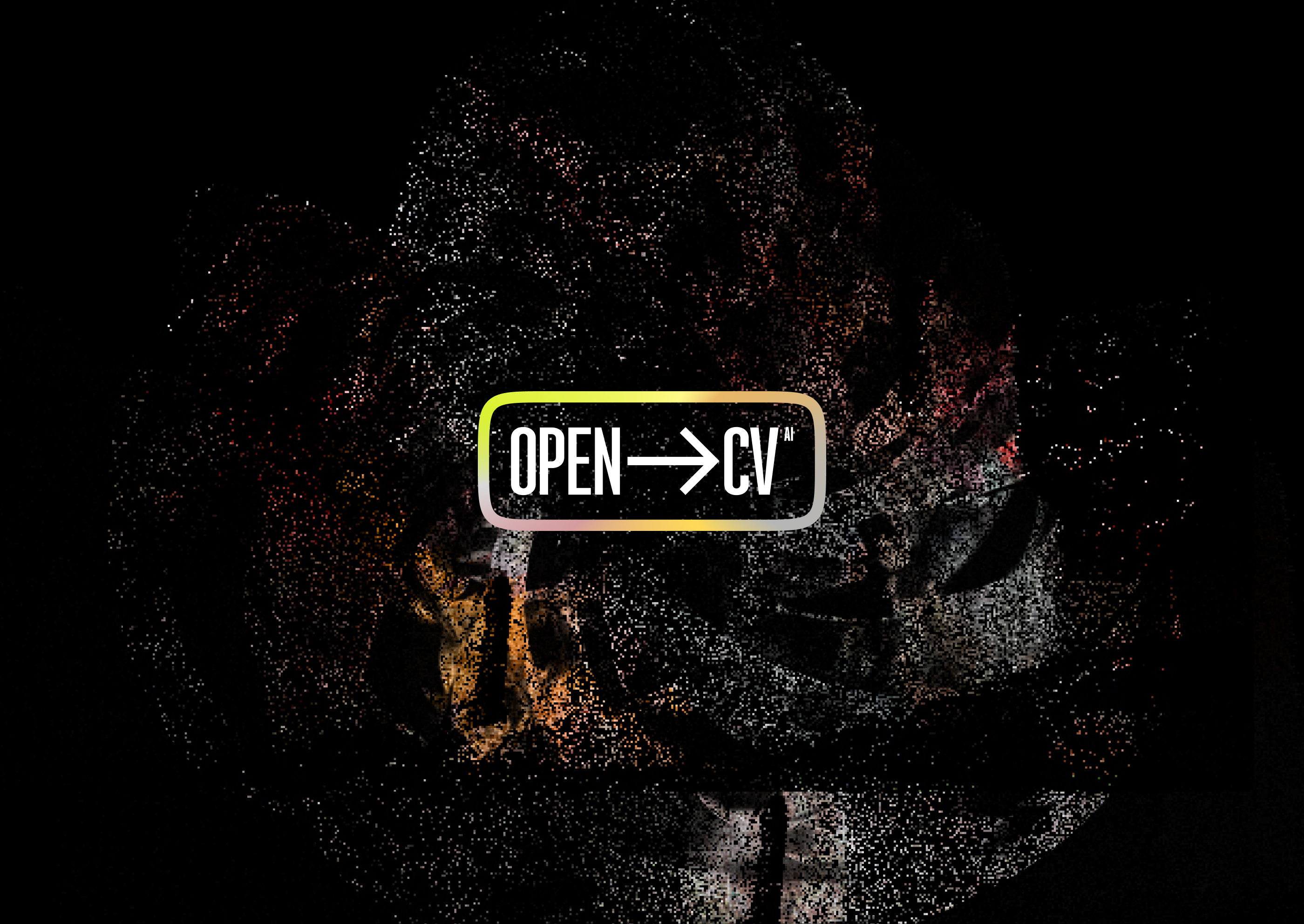
May 3, 2023
Our Consulting Process: Empowering Businesses with AI Solutions
At OpenCV.ai, we believe that computer vision (CV) has the potential to revolutionize businesses of all sizes, from ambitious startups to Fortune100 companies. Our for-profit arm leverages the same expertise that made OpenCV the most popular CV library in the world. In this post, we'll give you a glimpse into our consulting process, complete with timelines, and how it can help you harness the power of CV to transform your business.
READ MORE
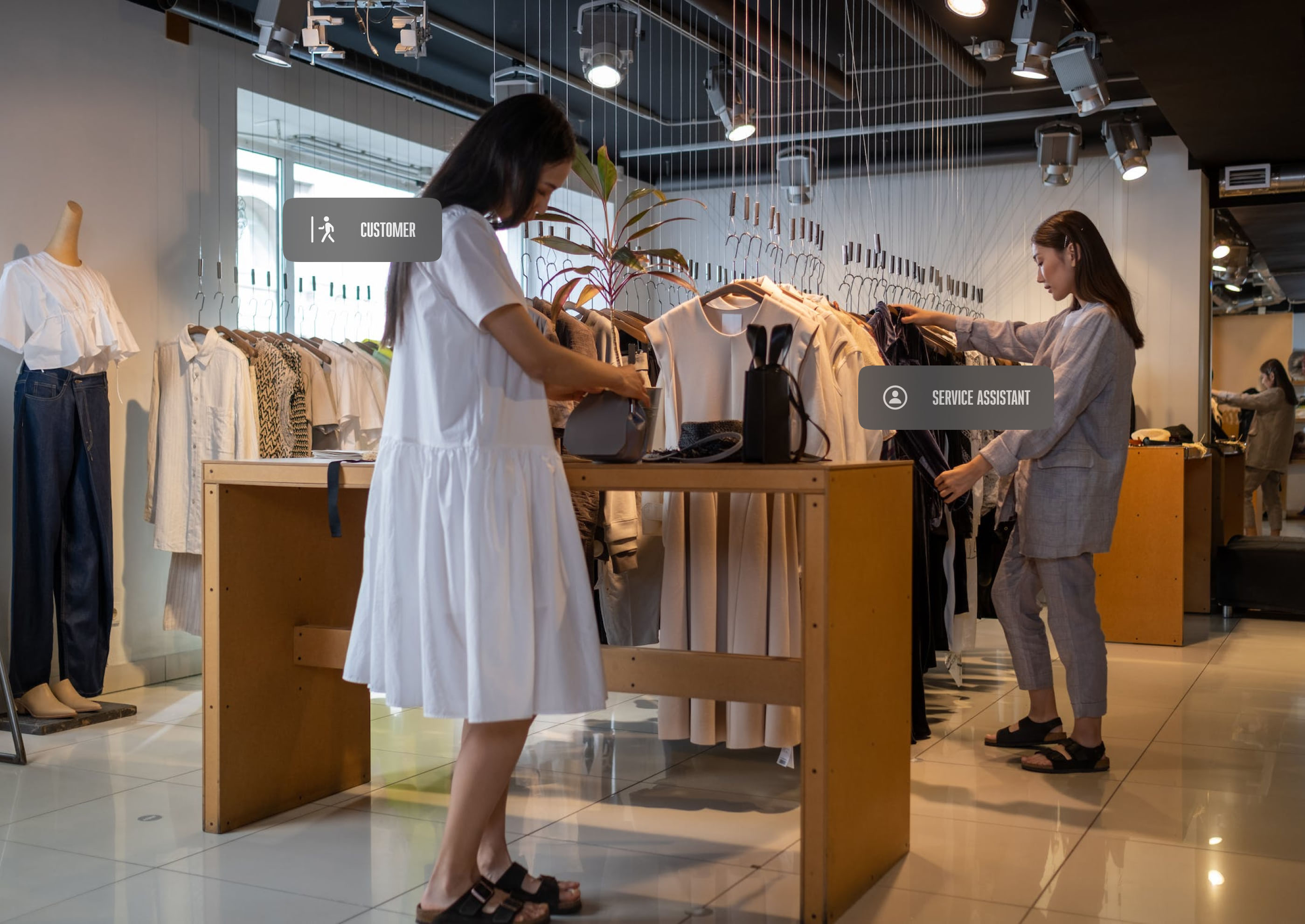
April 28, 2023
Applied AI #1. Real-Time Multi-Object Tracking in AI and CV
In today's deep learning era, AI and computer vision (CV) have enabled us to tackle complex challenges with remarkable speed and accuracy. In this article, we'll explore the world of multi-object tracking in CV and discuss its applications, challenges, and breakthroughs.
READ MORE